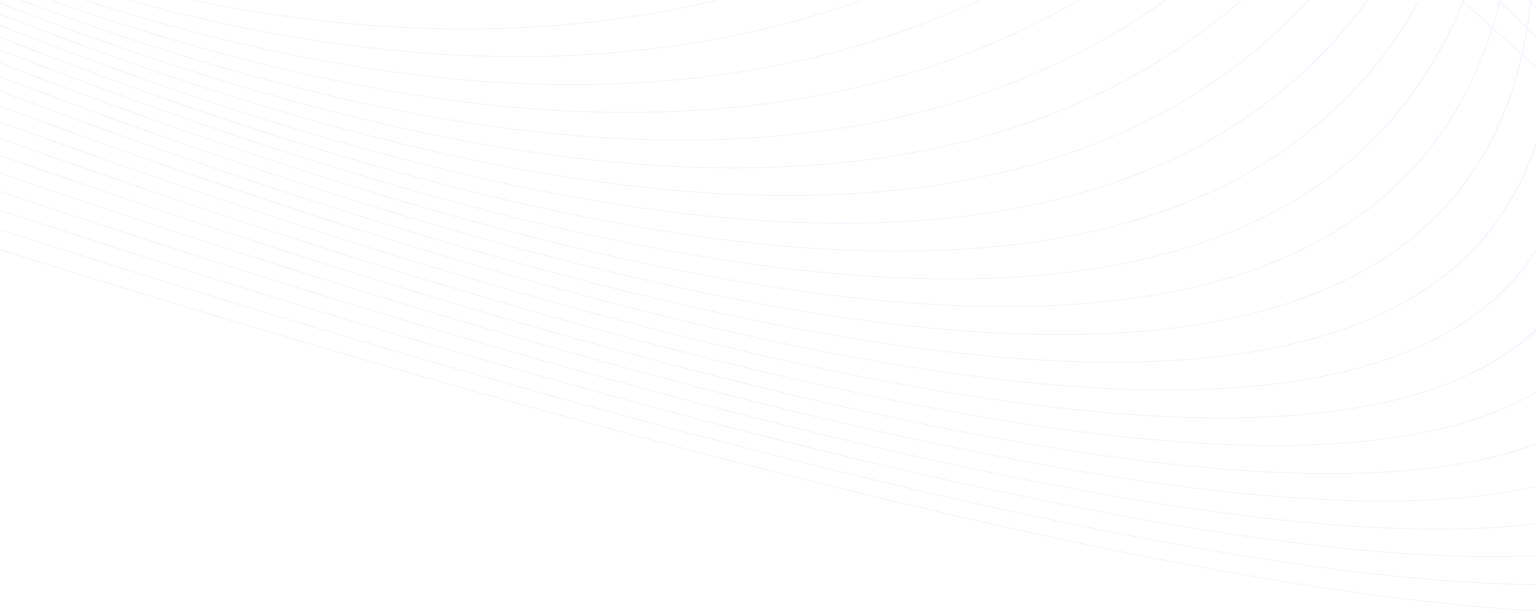
If you’re comparing data mesh vs data fabric, you’re on the cusp of making a transformational decision for your organization’s data. But choosing the right data management strategy is as challenging as it is vital.
Fortunately, you’ve come to the right place. In this article, we’ll explain and compare data mesh and data fabric: their key principles, pros and cons, and examples of companies that have successfully used each approach. You’ll come away empowered to make the best decision for your business needs.
What is Data Management?
Data management is the process of successfully and efficiently gathering, storing, organizing, preserving, safeguarding, and using data. Data management is now an integral part of daily operations in today's modern enterprises as data is increasingly recognized as a valuable resource that may foster business growth, innovation, and competitive advantage.
These are some of the factors that make data management crucial in contemporary businesses.
- Enhanced customer experience.
- Improved risk management.
- Improved operational efficiency.
- Better decision-making.
- Regulatory compliance.
While Data Mesh and Data Fabric are different approaches to managing data, they both aim to address the challenges of managing large amounts of data in complex organizational environments. Both approaches can be effective depending on the needs and goals of the organization.
This article aims to clarify the differences between data mesh and data fabric and provide guidance on which approach to use for your organization's data management needs.
What is Data Mesh?
Zhamak Dehghani, a principle consultant at Thoughtworks, presented a novel method for planning data architecture in big enterprises called "Data Mesh." It is a decentralized model that promotes the ownership of data by the domain teams that are most in tune with the business opportunities and problems.
Data is handled like a product in a Data Mesh architecture: owned, delivered, and consumed by domain teams. Each domain team is in charge of maintaining its own data pipelines, storage, and processing, as well as creating and distributing its own data products. With standardized interfaces and APIs, the data products are made available to various teams inside the business.
Data Mesh makes innovation, decision-making, and data processing more effective by decentralizing data ownership and making data products accessible throughout the enterprise. Data silos, a lack of confidence in the data, and the difficulty of maintaining and extending data pipelines are some of the problems that Data Mesh seeks to address. These problems are frequently encountered in traditional centralized data architectures.
Many businesses are beginning to organize their data architecture using the Data Mesh method, which has garnered significant support in the data community. It is still a new idea, though, and there are still arguments and conversations about how to really put it into practice and what its limitations are.
Key principles of data mesh
These principles aim to address some of the challenges of traditional centralized data architectures, such as data silos, lack of trust in data, and the complexity of managing and scaling data pipelines.
- Domain-oriented decentralized data ownership and architecture: The domain teams who are most in tune with the business possibilities and problems own the data in a data mesh architecture. These teams are in charge of running their own data processing, storage, and pipelines. This strategy promotes greater agility and quicker decision-making since the domain teams are able to adjust to shifting business requirements.
- Data as a product: Domain teams are considered to be the owners, suppliers, and consumers of data. This indicates that data must adhere to the same governance, lifespan, and quality criteria as any other product.
- Federated governance: Each domain team is in charge of managing its own data, and governance in a data mesh architecture is federated across the enterprise. To maintain data security, compliance, and quality, there are still centralized regulations and standards in place.
- Self-serve data infrastructure as a platform: Domain teams can control their own data pipelines, data storage, and data processing thanks to the self-serve platform design of the data infrastructure in a Data Mesh architecture. This makes it possible to use resources more effectively and eases the workload on central IT personnel.
Benefits and drawbacks of data mesh
The benefits of Data mesh include:
- Decentralized ownership.
- Data as a product.
- Self-serve data infrastructure.
- Federated governance.
Some drawbacks of data mesh include:
- Complex implementation.
- Higher operational costs.
- Potential for data duplication.
- Governance challenges.
Use cases and examples of organizations using data mesh
There are several use cases and companies using data mesh. Here are a few of them:
- Uber: Uber, a leading ride-sharing and transportation company, has adopted Data Mesh to improve their data processing and decision-making capabilities. They have decentralized their data ownership and established cross-functional data product teams that are responsible for their own data pipelines, data storage, and data processing.
- Thoughtworks: Thoughtworks, a global software consultancy, has implemented Data Mesh to manage its data infrastructure and processing needs. They have established data product teams that are responsible for their own data pipelines, data storage, and data processing.
- Zalando: Zalando, a leading fashion and lifestyle e-commerce company, has adopted Data Mesh to manage its complex data ecosystem. They have decentralized their data ownership and established cross-functional data product teams, each responsible for a specific domain of data.
What is Data Fabric?
Data Fabric is an approach to data management that aims to create a unified and integrated view of data across an organization, regardless of where the data is located or how it is stored. The term "Data Fabric" was coined by Gartner, a leading research and advisory company, to describe an emerging trend in data management.
A Data Fabric is a layer of software that sits between the various data sources and applications within an organization, providing a single interface for accessing and managing data. It enables organizations to bring together data from a wide range of sources, including databases, data warehouses, data lakes, and cloud storage, and make it available to users and applications consistently and seamlessly.
Data Fabric is designed to address some of the challenges associated with traditional data management approaches, such as data silos, data fragmentation, and data complexity. By providing a unified view of data across the organization, Data Fabric enables faster and more efficient data processing, better decision-making, and improved collaboration between different teams and departments.
Key principles of data fabric
- Data integration: the ability to integrate data from multiple sources, including structured and unstructured data.
- Data management: the ability to manage data across its entire lifecycle, from ingestion to archiving and deletion.
- Data governance: the ability to manage data security, privacy, compliance, and quality.
- Data discovery: the ability to discover and understand data assets across the organization.
- Data access: the ability to provide secure and controlled access to data for users and applications.
Benefits and drawbacks of data fabric
The benefits and drawbacks of data fabric are discussed below:
Benefits of data fabric include:
- Scalability: Data Fabric can easily scale up or down to meet changing business needs and data volumes.
- Data Integration: Data Fabric provides a unified view of data across multiple sources, enabling seamless integration and data sharing.
- Cost-Effective: Data Fabric can help reduce the cost of data management by eliminating data silos and reducing the complexity of data integration.
- Real-time Insights: Data Fabric enables real-time data access, processing, and analysis, which helps organizations make faster and more informed decisions.
- Agility: Data Fabric provides the flexibility to add new data sources, applications, and data services as needed, without disrupting the existing infrastructure.
Some drawbacks of data fabric include:
- Governance: Data Fabric can create governance challenges, as it requires a unified governance framework across multiple sources and applications.
- Data Quality: Ensuring data quality can be a challenge in Data Fabric, as it involves data from multiple sources with varying quality and standards.
- Complexity: Implementing Data Fabric can be complex, as it involves integrating multiple data sources, applications, and services.
- Security and Privacy Concerns: Data Fabric can pose security and privacy concerns, as it involves data sharing across multiple sources and organizations.
- Vendor Lock-in: Data Fabric may result in vendor lock-in, as it requires specialized tools and platforms that may not be interoperable with other technologies.
Use cases and examples of organizations using data fabric
- Visa: Visa, the global payment technology company, implemented Data Fabric to manage its vast amounts of transaction data across multiple platforms and geographies.
- Cisco: Cisco, the networking and communications company, implemented Data Fabric to improve its data analytics capabilities and drive business outcomes. With Data Fabric, Cisco has been able to integrate data from various sources, including sensors, devices, and applications, and analyze it in real time.
- Nordstrom: Nordstrom, the fashion retailer, implemented Data Fabric to improve its customer experience and drive sales. Data Fabric enables Nordstrom to analyze customer data from various sources, including in-store and online purchases, social media, and customer service interactions.
Data Fabric Vs. Data Mesh: Key Comparison
Feature | Data Mesh | Data Fabric |
---|---|---|
Data Access | Data is accessed through standardized APIs. | Data is accessed through a unified interface. |
Definition | A decentralized approach to data management where data is owned by individual domains and accessed through standardized APIs | A centralized approach to data management is where data is stored in a centralized repository and accessed through a unified interface. |
Data quality management | Each domain is responsible for the quality of its own data. | The central repository is responsible for the quality of all data. |
Data processing | Data processing is done at the domain level. | Data processing is done centrally. |
Data governance | Decentralized governance with each domain responsible for its own data governance. | Centralized governance with a central repository responsible for data governance. |
Architecture | Domain-oriented architecture with each domain owning and managing its own data. | Centralized architecture where data is owned and managed by a central repository. |
Scalability | Highly scalable due to the distributed nature of data management. | Limited scalability due to the centralized nature of data management. |
Complexity | Higher complexity due to the decentralized nature of data management. | Lower complexity due to the centralized nature of data management. |
Analysis of when to use data mesh vs. data fabric
The data mesh approach is well-suited for organizations with complex data ecosystems, where data is generated and consumed by multiple teams and applications. The data fabric approach is well-suited for organizations with simpler data ecosystems, where data is generated and consumed by a smaller number of teams and applications.
Considerations for implementing data mesh or data fabric in your organization
When deciding between data mesh and data fabric, it is important to consider several key factors:
- Organizational structure
- Data governance
- Data scalability
- Data security
- Data culture
Conclusion
In summary, data mesh and data fabric represent two different approaches to data management, each with its own strengths and weaknesses. The right data management approach will depend on a range of factors, including your organization's specific needs and goals, the size and complexity of your data, and the level of control you require over your data.
By carefully considering these factors and evaluating the pros and cons of different approaches, you can make an informed decision that will set your organization up for success in the years to come.
The key differences between them can be seen in their definition, scalability, complexity, architecture, data governance, data processing, and data quality management.
Whatever approach you choose, we designed Estuary Flow as a flexible real-time integration solution for your data architecture. Build your first pipeline for free today!
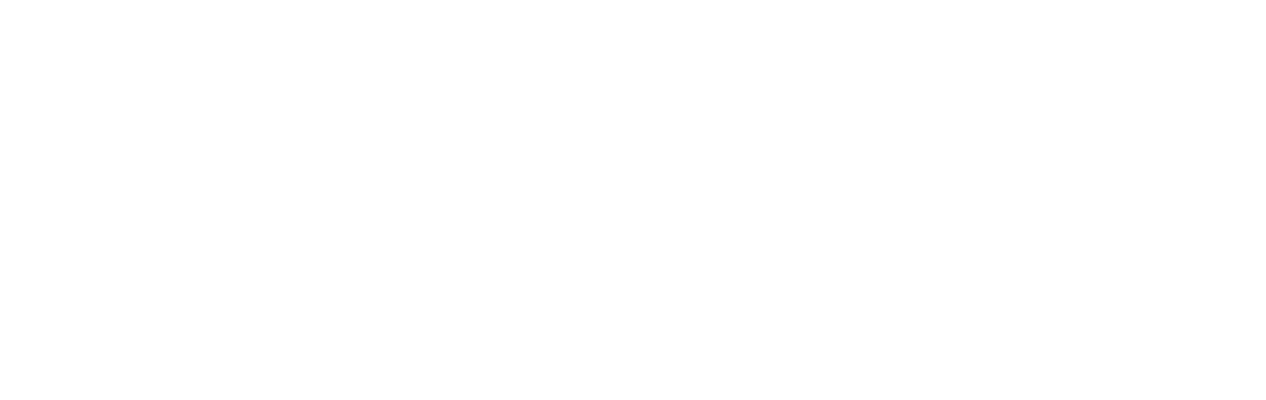
About the author
With over 15 years in data engineering, a seasoned expert in driving growth for early-stage data companies, focusing on strategies that attract customers and users. Extensive writing provides insights to help companies scale efficiently and effectively in an evolving data landscape.
Popular Articles
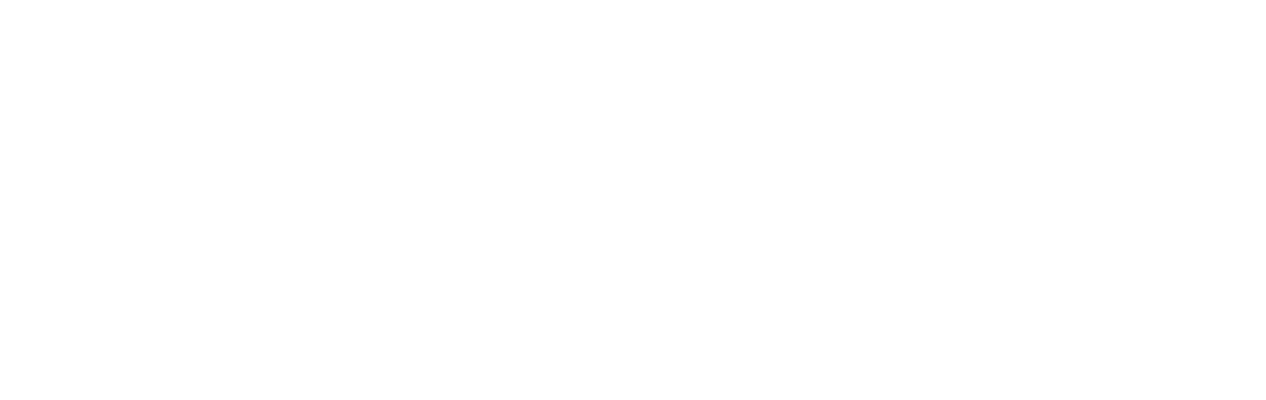