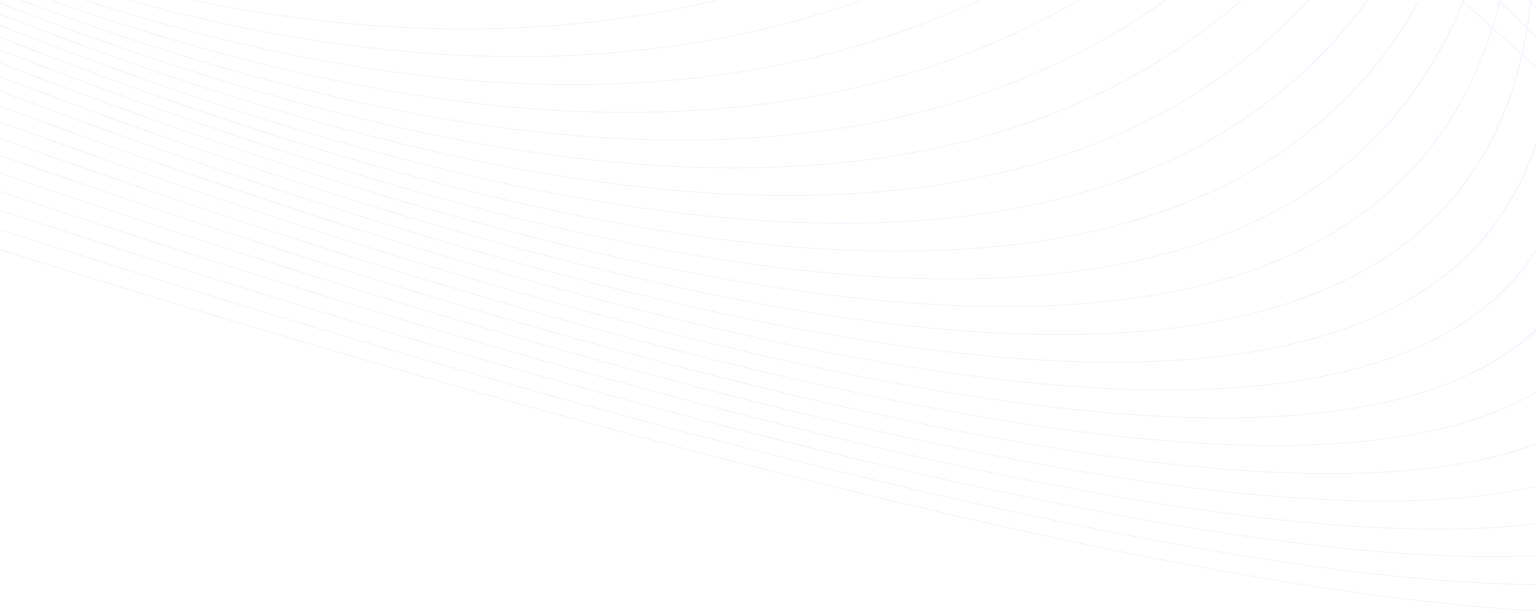
The biggest question in today’s data management landscape is how to tame the sprawling data ecosystem and transform it into a strategic asset. The answer lies in the power of effective data governance – an advanced framework that guides organizations in achieving data integrity, security, and value extraction on a scale never witnessed before.
As customer data and information continue to proliferate at a phenomenal pace, only those organizations that proactively embrace a comprehensive data governance framework will thrive in the face of complexity.
To make sure that yours is one of them, we have prepared this guide. Today, we will talk about data governance, its frameworks, and best practices. By the time you’re done reading this power-packed guide, you’ll have a complete understanding of data governance and know how you can implement it for maximum efficiency.
What Is Data Governance?
Data governance (DG) is an important process that ensures the availability, integrity, and security of data within an organization's systems. With stringent data privacy regulations and increasing dependence on data analytics, it's crucial to have reliable and consistent data that's safe from misuse.
At its core, DG involves a set of processes, roles, standards, and metrics. These elements work together to use information effectively in achieving organizational goals. By setting out clear processes and responsibilities, DG ensures high-quality, secure data usage across the board.
A solid DG strategy is especially crucial for organizations dealing with big data. It provides a framework to guide the business in establishing common processes and responsibilities. The strategy pinpoints critical data requiring stringent control and anticipates the benefits of these efforts.
What Is Not Data Governance (DG)?
Confusion often arises when distinguishing data governance from data management, master data management, and data stewardship. Here's how they differ:
Data Governance Vs Data Management
Data management oversees an organization's entire data lifecycle. While data governance forms a key part of this, it's not the whole picture. It ties into areas like data quality, security, metadata management, and data warehousing.
Data Governance Vs Master Data Management
Master data management (MDM) focuses on identifying and refining the quality of an organization's primary entities like customers or suppliers. It takes fragmented entity views and unifies them, a task that goes beyond data governance. However, successful MDM hinges on robust governance to define data models and detail data retention policies, among other responsibilities.
Data Governance Vs Data Stewardship
The goal of data stewardship is to keep the data correct, easy to find, and under control for the right people. It's about putting the strategic, organizational, and policy-related aspects of data governance into action. So while data stewards ensure data consistency with the governance plan and oversee data quality and compliance, their role is execution and operationalization, distinct from broader data governance.
The Scope Of Data Governance
Now let’s discuss the main components that define the breadth and depth of data governance.
- Quality assurance: Setting up and tracking rules to ensure and maintain data quality.
- Data protection: Ensuring the security and safety of all data, tracking who accesses it, and how it's used.
- Ownership identification: Tracking data ownership to ascertain responsibility for data integrity and security.
- Software selection: Picking suitable data governance tools that can address the wide-ranging needs of data governance effectively.
- Data understanding: Grasping the creation, usage, and access of organizational data, inclusive of metadata comprehension and data dictionary usage.
- Usage tracking: Maintaining an audit trail for data creation, reading, and deletion, with the capability to search the trail and spot unusual usage patterns.
- Workflow automation: Creating and automating workflows for data discovery, archiving, and deletion. This includes automating policy creation and application.
- Handling diverse data sources: Accommodating a variety of data assets and sources, from traditional databases and spreadsheets to emails, instant messages, videos, audio files, and other unstructured content.
- Metadata management: Constructing and managing data models and business glossaries, establishing custom attributes for data description, and setting up data validation and enrichment rules.
Now that you’re familiar with the basics of data governance, let’s build on this and discuss its frameworks and understand how you and your organization can benefit from it.
Understanding Data Governance Frameworks
A data governance framework sets a structured approach for managing, securing, and storing sensitive data in an organization. It begins with assigning strategic, tactical, and operational roles to ensure data privacy and compliance, driven by the organization's unique business drivers. These drivers help determine the necessary levels of control for different types of data.
A key aspect of a successful data governance framework is building trust in the data. Ensuring reliable, well-documented, and easily findable data within the organization achieves this. Equally important is the need to maintain security, confidentiality, and compliance with relevant regulations.
Establishing the data governance framework also depends on 3 essential components. First, it needs to have funding and backing from management. Second, user engagement is crucial as those who consume the data should understand and follow the governance rules. Last, a formal body, known as a data governance council, is required to define and enact the framework in the organization.
Types Of Data Governance Frameworks
3 types of data governance frameworks exist:
- Command and Control: Assigns a few employees as data stewards.
- Traditional: Appoints a larger voluntary group as data stewards with a few having additional duties as "critical data stewards".
- Non-Invasive: Recognizes individuals as data stewards based on their existing relationship with the data.
Each type of framework offers a different approach to stewardship and data management, thus suiting different organizational needs.
Essential Elements Of A Data Governance Framework
Let’s discuss the fundamental elements that constitute a successful data governance framework.
Personnel & Training
The heart of a data governance framework is the team, made up of data governance experts, data stewards, and other key staff from business and IT. They build and manage the processes that handle the company's data governance requirements. For this, the team needs to spend on education and training.
Strategic Planning For Data
Creating an enterprise data strategy is pivotal for successful data management. The strategy is a managerial document that outlines high-level data requirements and lays out a plan to achieve these goals.
Processes For Data Management
A robust data governance framework requires implementing key data processes. These include everything from tracking and resolving data issues to managing data exchange, maintaining quality control, and tracing data lineage.
Regulations For Data Protection
A data policy, comprising statements that articulate expectations and desired outcomes, is crucial to guide data-related behaviors at a business level. These policies are established via data governance initiatives and include aspects like regulatory compliance and outbound data sharing.
Data Standards And Rules
To ensure compliance with data policies, establish data standards and rules. These rules govern activities and assure adherence to data standards, impacting various areas like:
- Accessibility
- Classification
- Data definitions
- Domain development
- Metadata management
- Master and reference data
Safeguarding Data
Data security is a crucial component that involves protecting digital data from damaging factors and undesirable actions such as theft, hacking, or data breaches.
Technological Support
Automated data governance programs require a suite of technologies. Data governance software can simplify tasks like scanning databases, managing metadata, automating data stewardship processes, and enabling collaboration.
Performance Indicators & Metrics
To measure the impact of the data governance program, you should set quantifiable business metrics and KPIs. These measurements should be consistent over time to provide a reliable method to track the program's effectiveness.
Communication Plan
Effective communication about data governance activities is a critical aspect of a governance program. A sound communication plan outlines the objectives, targets, and tools for all communications, ensuring a unified message across the organization.
Integration & Socialization
Integrating data governance into the organization's rules, culture, structure, and operations is crucial for a successful framework. This continual process, termed data governance socialization, ensures that the organization adopts the new approach to managing data across all levels.
3 Approaches To Develop Data Governance Frameworks
Here are 3 distinct methodologies for developing data governance frameworks.
Centralized (Top-Down) Approach
In a centralized or top-down approach to data governance, having control over data is the most important factor to consider. It employs a small team of data professionals who use defined methodologies and best practices. The focus is on data modeling and governance before data is distributed to the rest of the organization for analytics.
However, the centralized approach faces scalability challenges. Only a small team, typically IT, has control over the data causing potential bottlenecks. As the demand for clean, uncompromised data from various business units increases, this approach struggles to keep pace.
Decentralized (Bottom-Up) Approach
In contrast, the decentralized or bottom-up approach emphasizes data access. It starts with raw data ingestion, followed by the creation of structures and implementation of data quality controls, security rules, and policies.
This approach is catalyzed by the advent of big data and is more scalable. However, it comes with its own set of challenges. As governance isn't the initial focus and data input is unrestricted, maintaining control over data can be difficult. This causes regulatory risks, stakeholder distrust, and increased data management costs.
Collaborative Approach
The collaborative approach is a modern data governance framework that balances control and access. It involves teamwork across the organization, recognizing that data ownership and curation should not be limited to a small group. This approach allows scalability without compromising trust in the data.
In the collaborative approach, principles for data curation are established. Subject matter experts from various business units serve as data stewards to maintain high data quality for the datasets they know best.
This approach engages everyone in the organization for turning raw data into a trusted, documented, and shareable enterprise data set. However, for heavily regulated data elements, a more controlled top-down approach may still be necessary. Therefore, the collaborative approach is used as a complement rather than as a replacement.
Now that we have a complete grasp of data governance frameworks, let's take a look at how they significantly influence the application and effectiveness of data governance principles and the broader implications of an effective data governance strategy.
5 Principles Of Data Governance
The principles of data governance act as guiding lights for establishing a sound data management strategy. They outline the fundamental values and concepts that underpin an effective governance framework.
Let's look into these principles to build a more secure and efficient data governance strategy.
Accountability
A cornerstone of data governance is accountability. Every department within an organization should take responsibility for data governance. Establish a data governance council to ensure responsibility is distributed across the organization. This council develops and implements data processes and policies consistently throughout the organization.
Normative Laws & Regulations
Establish uniform rules and regulations that are in line with relevant external laws to secure data. The data governance council, along with the data steward, should formulate these standardized rules and regulations. They serve as guidelines for all data usage scenarios within the organization.
Administration Of Data
Assign a data administrator to enforce data rules and regulations and ensure adherence to these standards regularly. The data administrator is accountable to the data council.
Data Quality Standards
Collaboratively, the data governance council and the data steward should set data quality standards. These standards help evaluate and document data quality, ensuring that everyone in the organization understands and adheres to these guidelines.
Transparency
All processes relating to data governance should be as transparent as possible with a detailed record of all relevant actions and procedures. This transparency ensures audit readiness and provides insights into data usage across the organization.
Knowing the data governance principles, let's now discuss how these foundational guidelines and standards are incorporated to derive the best data governance practices for effective data management.
6 Best Practices For Data Governance
Effective data governance requires clear guidelines. Here are some carefully-curated best practices based on proven methodologies and insights from industry leaders.
Start With The Basics
When implementing data governance, the first step is to assemble a dedicated team. This team will have the responsibility of understanding and managing the data within your organization.
Once the team is in place, they can proceed to create efficient processes for handling data. This involves defining and implementing policies, procedures, and guidelines for data management. These processes ensure that data is collected, stored, processed, and accessed in a consistent, secure, and reliable manner.
Showcase The Benefits
Leaders and stakeholders need to see the value of data governance. So, highlight how data governance can lead to increased revenue. Explain how it enhances customer experiences and provides accurate and timely information. Show how it can streamline operations and make your organization more efficient.
Measure Progress
Start by determining your 'baseline' or the current state of your data management. This will serve as a comparison point once you begin making changes. After you start your data governance practices, regularly measure how well you're doing. Use metrics to track your progress. This will help you see if your new processes are working or if they need adjustments.
Keep The Lines Of Communication Open
Communication is a key aspect of data governance. Everyone involved should know what's going on at all times. Regular updates about successes and setbacks ensure everyone is on the same page. This can also help to solve problems faster and celebrate successes together.
Plan For The Long-term
Data governance is not a one-time project. It's a continuous process that should be part of your organization's long-term strategy. Start with small projects to understand the process better. The learnings from these small projects can then be used to inform larger, more complex initiatives.
Define Roles Clearly
For a data governance program to succeed, everyone should know their roles and responsibilities. These roles include:
- Data users
- Data owners
- Data stewards
- Data managers
- Data governance board
- Data governance council
Each role has different tasks and responsibilities so make sure everyone knows what they're supposed to do.
5 Data Governance Frameworks
There are several tested data governance frameworks, let's briefly look into the most important ones.
DGI Framework
The Data Governance Institute (DGI) offers a framework that focuses on 10 critical components. They determine the goals, metrics, funding, controls, and operations of your data governance program. Key aspects of the DGI framework include:
- Implementing controls for risk management can be preventive or corrective.
- Outlining how the data governance program can boost revenue, optimize costs, and ensure resilience.
- Establishing a Data Governance Office (DGO) to manage and coordinate all data-related policies, standards, and records.
DAMA DMBOK Framework
The Data Management Body of Knowledge (DAMA DMBOK) envisions data governance as a wheel with governance at its hub and 9 knowledge areas surrounding it. Crucial components include:
- Metadata Management focuses on collecting, categorizing, and maintaining metadata.
- Data Development involves designing, implementing, and maintaining data storage elements.
- Data Architecture Management refers to the structure of data and its connection with applications.
McKinsey Framework
McKinsey's data governance framework revolves around redesigning the entire organization to ensure successful data governance. The main elements of this framework include:
- Domain-based roles for daily execution of the data governance program.
- A Data Management Office (DMO) defines policies and standards while training and guiding data leaders.
- A data council to oversee the strategic direction of the program, review progress, authorize funding, and resolve issues.
Eckerson Framework
Eckerson Group's framework includes 6 layers and 39 components that emphasize people's role in data governance. Its main aspects are:
- A culture that promotes collaboration, data democratization, and transparency.
- Goals and standards that explain the reasons and methods for implementing a data governance program.
- Processes designed to meet the objectives of data governance initiatives, like ensuring data quality and cataloging metadata.
Deloitte Framework
Deloitte believes that effective data governance maximizes the value of data for operational effectiveness, decision-making, and regulatory requirements while minimizing risks associated with poor data management. Its framework highlights:
- Processes that outline data creation, modification, and maintenance.
- Organizations determining data governance roles and responsibilities.
- Policies and principles that guide data governance and data management.
- Governance controls define the metrics to measure data governance effectiveness.
- Tools and technologies that map out tooling, modeling, and data architecture implementation.
Estuary Flow - A Robust Solution For Effective Data Governance
Estuary Flow provides an effective solution for data governance, ensuring the availability, usability, integrity, and security of enterprise data.
Let's look into how Estuary's features can contribute to Data Governance:
Data Capture
Estuary's ability to capture data from various sources, including databases and SaaS applications, ensures that all relevant data is included in the governance framework. This contributes to the completeness and accuracy of the data being governed.
Transformations & Materializations
The platform's powerful transformation capabilities allow for the standardization and normalization of data which are key aspects of data governance. Materializations, or the maintenance of low-latency views across systems, ensure that data is consistently represented across the organization.
Extensibility & Built-in Testing
Estuary's extensibility and built-in testing features ensure that data governance policies can be consistently applied across all data sources and that the data's integrity is maintained.
Scaling Feature
The scaling feature helps in the application of that data governance policies at scale, across large volumes of data.
Monitoring & Schema Inference
Estuary's live reporting and monitoring features help in effectively enforcing data governance policies and ensure that any issues are quickly identified and addressed.
Conclusion
Data governance is all about creating a culture of accountability and transparency around data where every stakeholder understands their role and responsibility. A robust data governance framework helps leverage data effectively. By following principles and best practices, you can establish a data governance strategy that ensures data integrity, security, and usability.
To effectively implement your data governance strategy, leverage our DataOps tool, Estuary Flow. With its robust data capture, transformation, and monitoring capabilities, Estuary can help streamline your data governance processes, ensuring data integrity and usability at scale. Sign up for free and explore its many benefits or contact our team to discuss your specific needs.
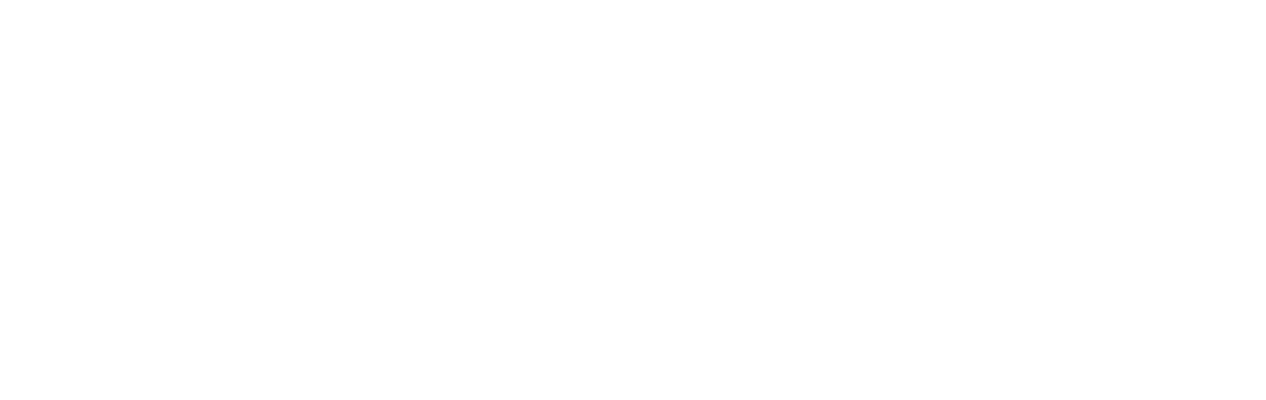
About the author
With over 15 years in data engineering, a seasoned expert in driving growth for early-stage data companies, focusing on strategies that attract customers and users. Extensive writing provides insights to help companies scale efficiently and effectively in an evolving data landscape.
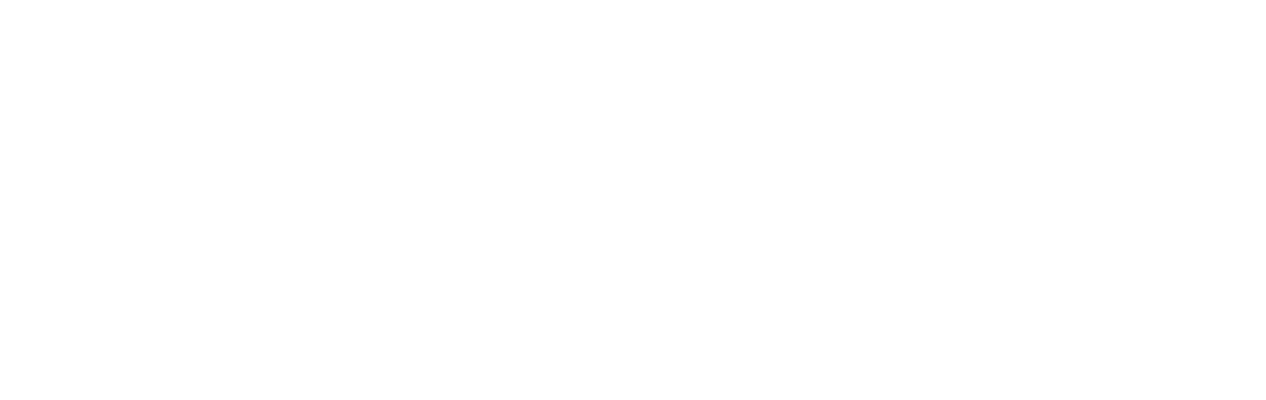