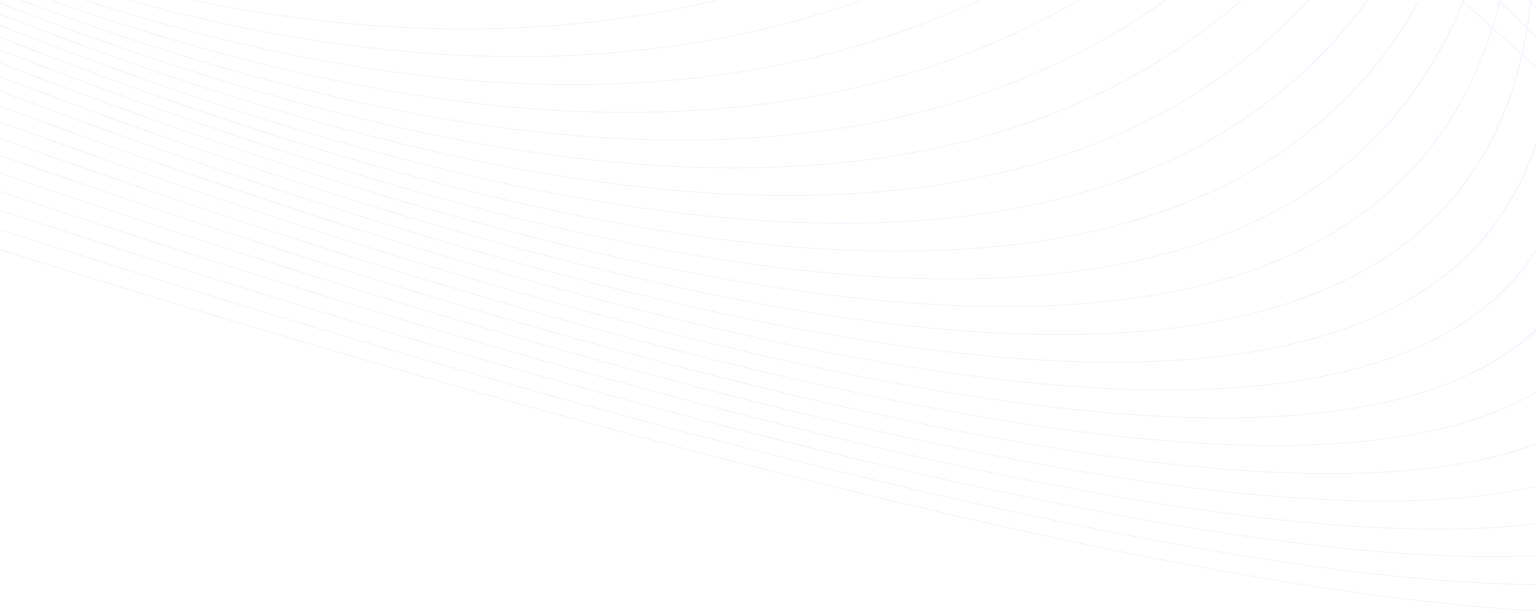
Data for all: Why data democratization matters at every scale
It’s vital to avoid data-related power imbalances among individuals, teams, and entire businesses. But data democracy takes work.
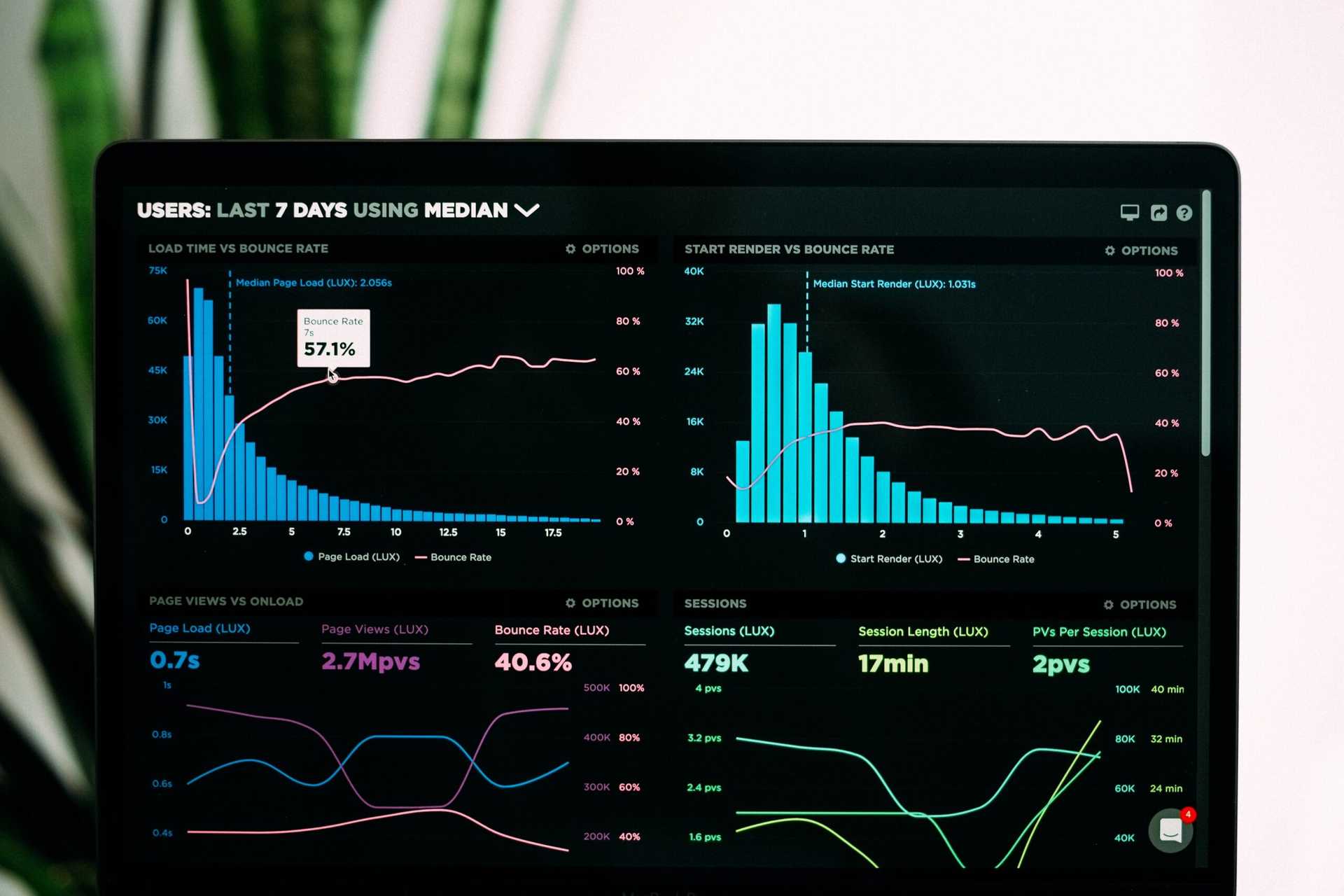
Data has become a vital resource across companies and teams. And as with most vital resources, it takes work to avoid power imbalance and unequal access.
That’s why the idea of data democratization is getting so much attention.
In this article, we’ll break down what data democratization is and why it matters now more than ever. We’ll talk about how it shows up on the scale of entire businesses, as well as between individuals in the same organization. And we’ll finish with some tips that’ll help you (or your team) pick the right tools to make data democracy a reality.
What is data democratization?
Data democratization is the access to the power of data without a lot of technical resources.
Let’s unpack that.
Data democratization is not simply access to data.
It’s one thing to have access to the raw data within an organization, and quite another to use it in the way you envision.
Having access to the power of data means having access to both the data and an appropriate suite of tools. These tools should complement the user’s skills and technical level, empowering them to extract value from the data.
Data democratization lowers the technical barrier around data.
It’s far too familiar of a problem: smart people fall short of their goals because they lack a specific type of technical expertise, and as a result, data-driven insight sits just out of reach.
This applies just as much to entire businesses (say, a growing organization with a small engineering team) as it does to individuals (like a marketing strategist who knows how to ask the right questions but not how to code).
Democratizing data is the act of intentionally removing barriers like these.
Why democratizing data matters in the 2020s
It’s not a coincidence that data democratization has become a much more popular topic in the past few years than ever before. There are two main reasons.
- With older technologies, data democratization wasn’t possible — or particularly compelling.
- Data has become mission-critical for just about every department of a modern organization.
Of course, these two points are related, and both are driven by the rapid evolution of data technology.
Siloed-off data tended by a small, specialized team is a paradigm we see frequently today. But it’s an outdated paradigm defined by the technological limits of a bygone period.
Think about it: in the early days of database technology, both compute and storage were expensive and unwieldy to manage. It simply didn’t make sense to consume limited resources — time and energy on your in-house servers — in the effort to supercharge every aspect of your business with data.
Data was used where it was absolutely required, and that was all. A small, highly specialized team was sufficient to manage its entire lifecycle.
What’s more, as a business, you didn’t need to worry about giving every team data superpowers. There wasn’t pressure in the market to do so. You could get by just fine doing sales and marketing, or making day-to-day business decisions the old-fashioned way. That is, you’d really on pre-packaged reports of older data, or simple human intuition.
Today, we’re in the midst of a Cambrian explosion of data technologies and methodologies. Most infrastructure has been cloud-based for years. We have innumerable UI-forward data visualization and SaaS tools. And we can integrate it all with the help of sophisticated data pipelining, workflow management, and visibility platforms — if we are up to the challenge of setting everything up correctly.
That is to say, with the right tools in place, there’s no reason for data to be siloed anymore. There is no reason for professionals who use data but don’t specialize in it to feel confused or disempowered.
It goes beyond that, too — from an organizational standpoint, it’s bad business for data stakeholders to feel confused or disempowered. (You can also argue that on the scale of our society, we’re all data stakeholders, which makes equity in data even more urgent.)
Today, you’d be hard-pressed to name an industry, discipline, or societal issue in which data-driven decision making is not expected. Far from the quarterly reports of bygone days, daily actions need to be informed by fresh data. The acceptable latency period is shortening, and true real-time data is gaining traction across a broader swath of use cases.
Failing to put data to work means companies can lose their edge to the competition. To do this well in every corner of every business, all stakeholders need to get more involved with data.
Data democratization for small and medium businesses
As mentioned above, data democratization can be thought of at the scale of the business, and at the scale of the individual. Let’s start with the business.
Large, data-centric tech companies will always have an edge over small and medium businesses (SMBs) when it comes to data technology. With huge data engineering teams, it’s only natural.
The problem is that technology is advancing at an unprecedented pace, and SMBs still struggle to implement new patterns of data use and infrastructure. As a result, the gulf between them and large enterprises continuously widens.
Let’s use change data capture as an example. There are multiple open-source options, for example, Debezium, an independent project; and DBLog, which was built as Netflix’s in-house framework. Still, self-hosting an open-source platform isn’t easy, and many SMBs decide that their current data infrastructure is “good enough,” focusing their limited engineering time elsewhere.
This isn’t just true of data; it’s a universal problem in the adoption of digital tools. A Deloitte survey of US small businesses found that “digitally advanced businesses” — defined in part as businesses that use website data to analyze trends and inform decisions — were more likely to rate ‘having no time to learn digital tools’ or ‘our business’s staff have inadequate digital skills’ as their top barrier to further advancement.
In other words, as small businesses try to grow past a basic level of digital proficiency, they hit a wall. Where data tech is concerned, this can be crippling.
In an ideal world, data democracy for SMBs should look like:
- Data tech innovations should be available for adoption at all business scales, either through open-sourcing or in the form of managed or SaaS platforms. Ideally, both options should be available.
- Though it will continue to exist, the lag in adopting new technologies between enterprises and SMBs should shorten.
Data democratization amongst teams
The other level where we need data democratization — and the one you’re more likely to have heard discussed — is between and within teams of a single organization.
We’ve already talked about the older organizational data paradigm and some of its common problems. These include:
- Data is stored in a central, hard-to-access monolith, and is managed by a team of data engineers. There’s a divide between the data experts and other teams that consume data, which often leads to misunderstanding.
- Data engineers spend much of their time performing repetitive workflows to keep the central monolith from breaking.
- Various teams across the company want data to inform their work, but fall short for many reasons which include:
- Not having access to data, or not having the skills required for access
- Not feeling like they can trust the data
- Lacking skills or analytics tools to extract answers from data
- Feeling that data experts are too busy to help them
In an ideal world, data democracy within a company should look like:
- All teams have the access, tools, and knowledge necessary to meet their specific data needs.
- Data is transparent, clean, and well-documented, which creates trust.
- Each team that uses data has full ownership of its specific data workflow.
There are various ways to think about (and implement) this, one of the most popular of which is data mesh and the idea of data as a product.
Different teams across a company need data that’s clean, accurate, available, and ready to be put to work in a specific way. This is referred to as a data product. We can give each team full ownership of its own data product lifecycle by breaking down the data monolith and creating distributed infrastructure, otherwise known as a data mesh.
Most importantly, this shift requires a change in data culture and more collaboration between data engineers and other teams that require data products. When done right, it can make everyone’s job easier.
How to choose data democratization tools
Of course, changes in organizational culture and data architecture will only get you so far toward data democratization. You also need a well-architected data stack and tools that match each stakeholder’s requirements and skill levels.
Here are a few tips:
- Start with identifying the data products you need, and then work backwards. Data product thinking is a helpful framework to outline data needs in different areas of an organization. What teams do you have? Who are the people on these teams? How will they, ideally, use data? What are the minimum requirements to allow this?
- Choose best-in-class tools for each task. Plenty of data tools advertise themselves as a panacea. It’s appealing to minimize the components that interact with your infrastructure. But realistically, tools that claim to do everything won’t do everything well, and they certainly won’t work for all teams. Focus on what each team actually needs to do its job without compromising.
- Pick a strong backbone. Even for deconstructed monoliths, you’ll always need centralized infrastructure to keep things in sync. Pick a data integration tool that’s customizable but reliable, and stands up to your requirements for data volume and timeliness. (Estuary’s platform, Flow, for instance, is designed for real-time integration).
- Embrace education as a tool. For any of this to work, data literacy is vital for all team members, even — perhaps especially — those with less technical background. Workshops, events, and reading materials can help. So can opening up avenues of collaboration between data engineers and other teams.
At Estuary, we’re dedicated to democratizing access to real-time data.
More specifically, the platform, Flow, is designed to unite data teams of diverse skills around flexible, scalable, real-time data pipelines.
Try Flow for free, connect with our team on LinkedIn or Slack, or check out our open code.
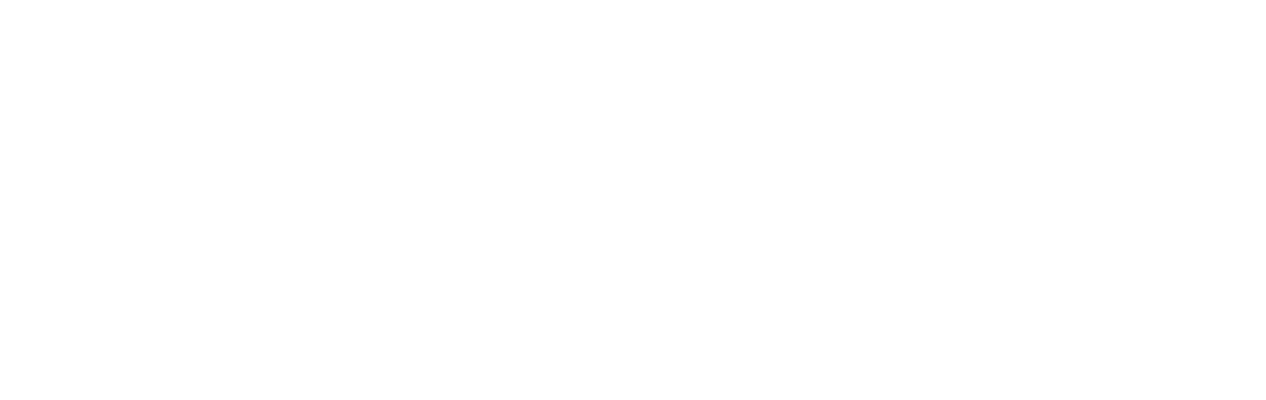
Author
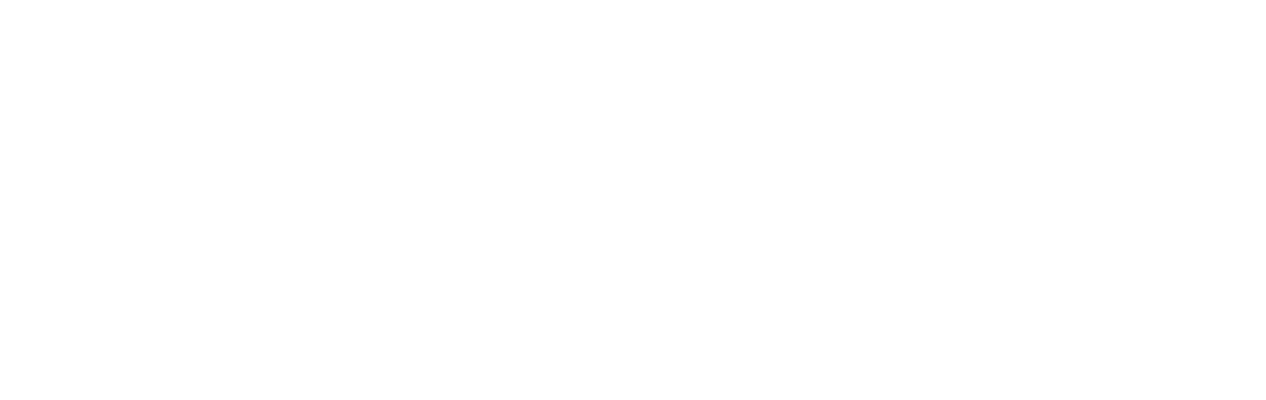