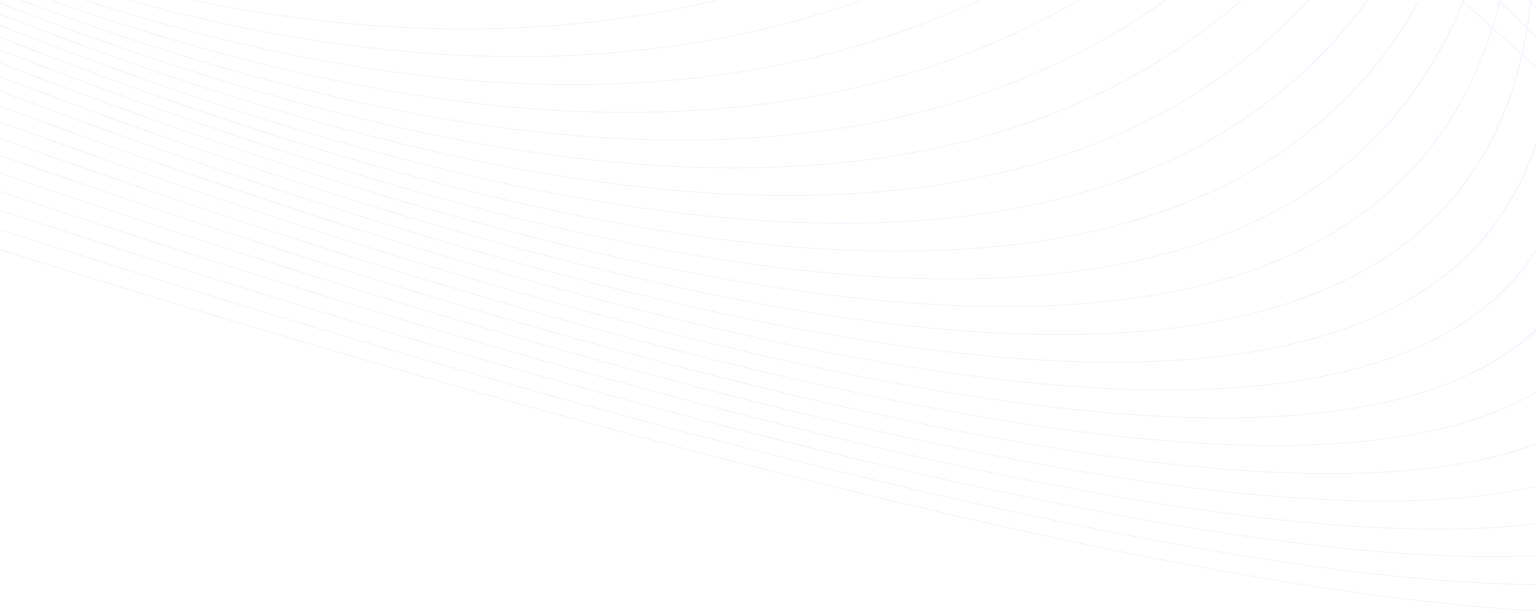
Microsoft SQL Server is a powerful OLTP system — but it wasn’t designed for scalable analytics or data lakes. Querying historical data or powering modern ML/BI use cases often leads to painful tradeoffs: slow batch jobs, costly compute, or limited scalability.
Apache Iceberg solves this. It’s the open table format built for scalable, fast, and flexible analytics across engines like Spark, Trino, and Flink.
But here’s the problem: moving real-time data from SQL Server to Iceberg is usually complex. Think scripts, Spark jobs, or fragile connectors.
Estuary Flow fixes this.
In this guide, we’ll show you how to build a real-time, zero-code pipeline from SQL Server to Apache Iceberg — using Estuary Flow.
Why Stream SQL Server to Apache Iceberg?
SQL Server is powerful for transactional workloads — but it wasn’t built for analytics at scale.
As data volumes grow, teams often struggle with:
- Query bottlenecks on live OLTP systems
- Expensive compute for historical analytics
- Limited compatibility with modern data platforms
That’s where Apache Iceberg comes in.
Iceberg is a high-performance, open table format that brings:
- Schema evolution without full rewrites
- Time travel and versioned data
- Compatibility with Spark, Trino, Flink & more
- Efficient columnar storage for petabyte-scale analytics
By syncing SQL Server to Iceberg in real time, you get the best of both worlds:
- Keep transactional performance fast in SQL Server
- Power analytics, ML, and BI at scale with Iceberg
Real-Time SQL Server to Iceberg with Estuary Flow
Estuary Flow is a real-time data integration platform that lets you:
- Capture CDC (Change Data Capture) from SQL Server
- Transform data with SQL or TypeScript (optional)
- Materialize to Iceberg tables using a REST catalog
Prerequisites
Before you begin:
- A free Estuary Flow account
- A SQL Server database with:
- CDC enabled on target tables
- A user with VIEW DATABASE STATE and SELECT permissions
- An S3 bucket + REST Catalog for Iceberg
- An AWS EMR Serverless application with the Spark runtime
- AWS credentials (access key & secret)
Step-by-Step: SQL Server to Iceberg with Estuary Flow
Step 1: Set Up SQL Server as the Source
- Go to Sources → + New Capture in the Estuary dashboard
- Select the SQL Server connector
- Provide the connection details:
- Address: <host>:1433
- Database: Your target DB
- Username/password: With CDC + SELECT permissions
- Choose your tables and specify primary keys if needed
- Click Next → Save and Publish
👉 Estuary will start capturing inserts, updates, and deletes in real time using CDC.
Step 2: Materialize to Apache Iceberg
- After capture, click Materialize Collections
- Search for and select the Apache Iceberg connector
- The Apache Iceberg connector can merge CDC updates while the Amazon S3 Iceberg connector instead relies on delta updates, which doesn’t reduce changes to your data
- Fill in your destination config:
- URL: base URL for the REST catalog
- Warehouse
- Namespace (e.g. sql_server_sync)
- Catalog authentication: OAuth 2.0 credentials or AWS SigV4 authentication
- Compute details: EMR application ID, S3 bucket, and access credentials
- Map your collections to Iceberg table names in the Source Collections section
- Click Next → Save and Publish
Estuary will batch CDC updates, convert to Parquet, and stream to your Iceberg tables — all in real time.
Advanced Options
Estuary Flow also supports:
- Delta Updates: Skip table queries, write faster (great for large-scale inserts)
- Backfill + CDC: Load historical rows, then stream new ones continuously
- Transformations: Filter, rename, or enrich data in-flight using SQL or TypeScript
- Scheduling: Control sync intervals (as low as 1 minute)
SQL Server to Apache Iceberg: Estuary Flow vs Manual Pipelines
Comparing Estuary Flow with traditional Spark-based or script-heavy pipelines reveals a major gap in simplicity, latency, and support for Iceberg — a modern open table format.
Feature | Estuary Flow | Custom Spark / Scripts |
Real-time CDC | Yes | Manual or slow |
Iceberg integration | Native | Complex setup |
No-code setup | Yes | Dev heavy |
Schema evolution | Auto | Manual |
Built-in reliability | Retries + checkpoints | DIY |
Setup time | Minutes | Hours or days |
Use Cases: SQL Server to Iceberg
Scalable Analytics
Run complex joins, aggregations, or time-travel queries on years of data — without hitting SQL Server.
ML Feature Stores
Sync operational data to Iceberg to train and serve real-time ML models.
BI Dashboards
Query streaming tables in Spark or Trino without stressing your primary database.
Compliance & Auditing
Store every change in Iceberg for secure, queryable historical records.
Final Thoughts: Real-Time SQL Server to Iceberg, Simplified
Modern analytics demands real-time data, flexible schemas, and scalable storage. But traditional ETL pipelines make syncing SQL Server to a data lake… painful.
Estuary Flow changes that.
With Estuary, you can stream every insert, update, and delete from SQL Server to Apache Iceberg — in minutes, with no code, and at massive scale.
- Real-time CDC
- Built-in Iceberg support
- Secure, fault-tolerant, and production-ready
Ready to modernize your SQL Server data strategy? Start streaming to Iceberg with Estuary Flow →
FAQ: SQL Server to Iceberg Integration
1. Does SQL Server natively support Apache Iceberg?
No. SQL Server doesn’t have native support for Iceberg. You need an external pipeline to sync your data into Iceberg-compatible storage. Estuary Flow bridges this gap by capturing change events (CDC) and streaming them directly into Iceberg tables with zero code.
2. Can I migrate historical and real-time SQL Server data into Iceberg?
Yes — Estuary Flow supports backfill + CDC. This means you can capture all existing records in your SQL Server tables and continue syncing new inserts, updates, and deletes in real time.
3. What if my SQL Server tables don’t have primary keys?
If your table lacks a primary key, Estuary allows you to manually define one during setup. This is required because Iceberg (and Flow collections) need a unique key to track data changes reliably.
4. Do I need Spark or Kafka to build this pipeline?
No. With Estuary Flow, there’s no need to manage Spark clusters, Kafka topics, or Airflow DAGs. The platform handles streaming, transformations, and Iceberg ingestion — all with an intuitive UI and pre-built connectors.
Related Resources
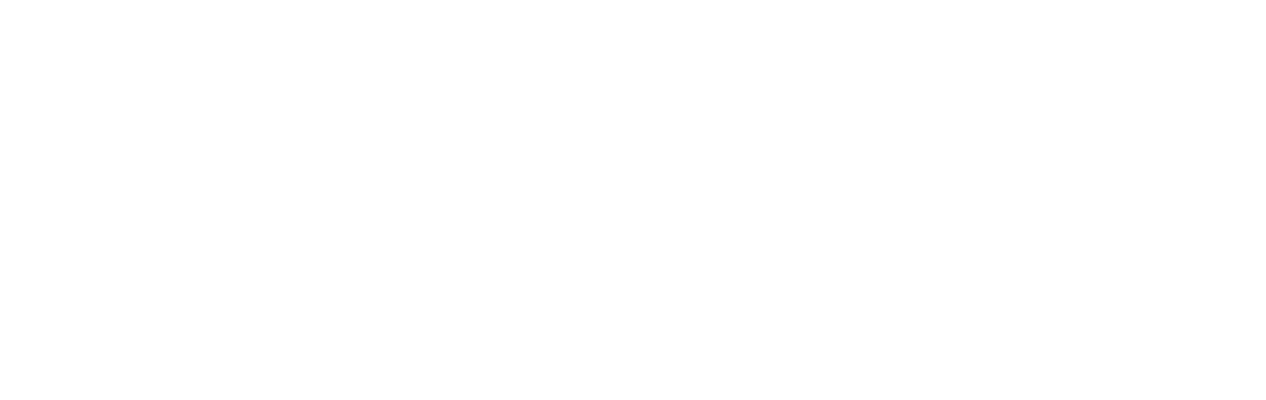
About the author
Dani is a data professional with a rich background in data engineering and real-time data platforms. At Estuary, Daniel focuses on promoting cutting-edge streaming solutions, helping to bridge the gap between technical innovation and developer adoption. With deep expertise in cloud-native and streaming technologies, Dani has successfully supported startups and enterprises in building robust data solutions.
Popular Articles
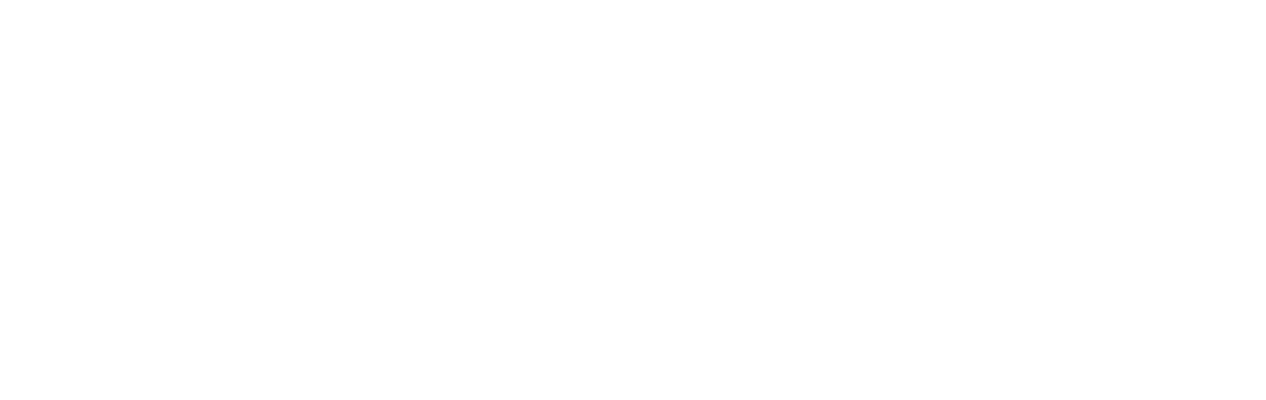