Fivetran VS Rivery
Read this detailed 2025 comparison of Fivetran vs Rivery. Understand their key differences, core features, and pricing to choose the right platform for your data integration needs.
View all comparisons
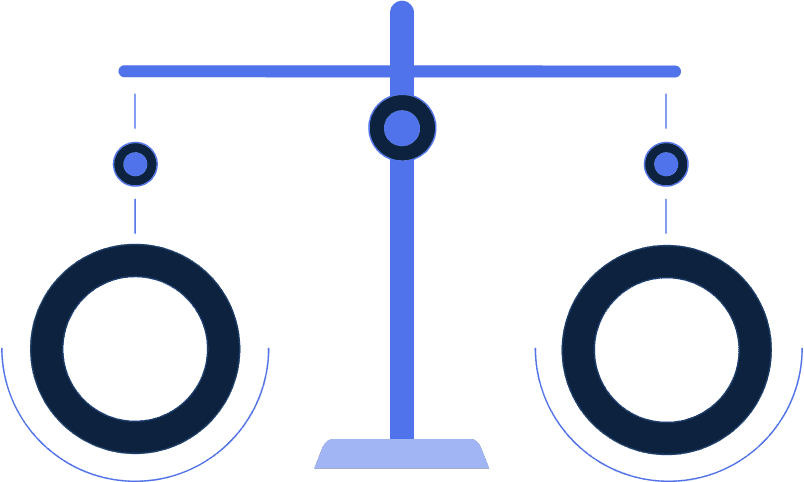

Introduction
Do you need to load a cloud data warehouse? Synchronize data in real-time across apps or databases? Support real-time analytics? Use generative AI?
This guide is designed to help you compare Fivetran vs Rivery across nearly 40 criteria for these use cases and more, and choose the best option for you based on your current and future needs.
Comparison Matrix: Fivetran vs Rivery vs Estuary Flow
![]() | ![]() | ![]() | |||||||||||||||||||||||||||||||||||||||||||||||||||||
---|---|---|---|---|---|---|---|---|---|---|---|---|---|---|---|---|---|---|---|---|---|---|---|---|---|---|---|---|---|---|---|---|---|---|---|---|---|---|---|---|---|---|---|---|---|---|---|---|---|---|---|---|---|---|---|
| |||||||||||||||||||||||||||||||||||||||||||||||||||||||
| |||||||||||||||||||||||||||||||||||||||||||||||||||||||
| |||||||||||||||||||||||||||||||||||||||||||||||||||||||
| |||||||||||||||||||||||||||||||||||||||||||||||||||||||
| |||||||||||||||||||||||||||||||||||||||||||||||||||||||
|
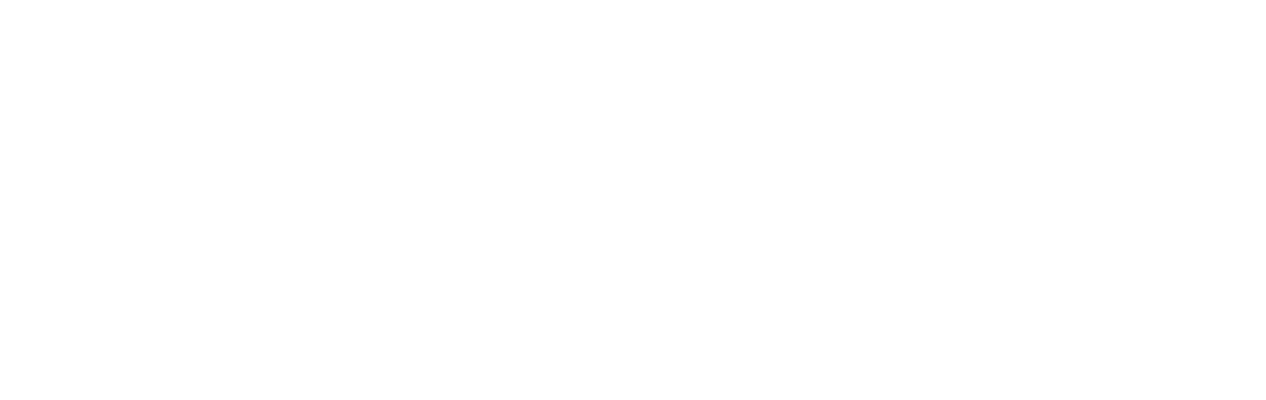
Fivetran
Fivetran was founded in 2012 by data scientists who wanted an integrated stack to capture and analyze data. The name was a play on Fortran and meant to refer to a programming language for big data. After a few years the focus shifted to providing just the data integration part because that’s what so many prospects wanted. Fivetran was designed as an ELT (Extract, Load, and Transform) architecture because in data science you might not yet know how you want to process your data, and so would want to store the raw data.
In 2018, Fivetran raised their series A, and then added more transformation capabilities in 2020 when it released Data Build Tool (dbt) support. That year Fivetran also started to support CDC. Fivetran has since continued to invest more in CDC with its HVR acquisition.
Fivetran’s design worked well for many companies adopting cloud data warehouses starting a decade ago. While all ETL vendors also supported “EL” and it was occasionally used that way, Fivetran was cloud-native, which helped make it much easier to use. The “EL” is mostly configured, not coded, and the transformations are built on dbt core (SQL and Jinja), which many data engineers are comfortable using.
Pros
- Ease of Use: Fivetran is a modern SaaS ELT platform with an easy-to-use UI, especially in comparison to more traditional ETL tools. It allows you to set up a data pipeline without coding.
- Pre-built Connectors: Fivetran offers nearly 300 native connectors and an additional 300+ “lite” connectors based on APIs.
- Scalability: Fivetran is known for scaling better than many of its competitors.
- Integration with dbt: dbt core is integrated into the Fivetran platform.
- Focus on replication: Fivetran is good at data extraction and loading (EL), even if it is batch only, making it a strong choice if your primary goal is to efficiently move data into your warehouse for analysis.
- Advanced schema evolution: Fivetran and Estuary are the two leading vendors with support for automating how changes in sources are passed through to destinations.
Cons
- Latency: While Fivetran uses change data capture at the source, it is batch CDC, not streaming. Enterprise-level is guaranteed to be 15 minutes of latency. Business critical is 1 minute of latency, but costs more than 2x the standard edition. Its ELT architecture can also be slowed down by the target load and transformation times.
- Costs: Fivetran’s high vendor costs can become an issue, as they have been 5x the cost of Estuary as stated by customers. Fivetran costs are based on monthly active rows (MAR) that change at least once per month. This may seem low, but for several reasons (see below and the pricing section) it can quickly add up.
- Unpredictable costs: Another major reason for high costs is that MARs are based on Fivetran’s internal representation of rows, not rows as you see them in the source.
For some data sources you have to extract all the data across tables, which can mean many more rows. Fivetran also converts data from non-relational sources such as SaaS apps into highly normalized relational data. Both make MARs and costs unexpectedly soar. This also does not account for the initial load where all rows count. - Reliability: Another complaint against Fivetran is reliability. Customers have struggled with a combination of alerts of load failures, and subsequent support calls that result in a longer time to resolution. There have been several complaints about reliability with MySQL and Postgres CDC, which is due in part because Fivetran uses batch CDC. Fivetran also had a 2.5 day outage in 2022. Make sure you understand Fivetran’s current SLA in detail. Fivetran has had an “allowed downtime interval” of 12 hours before downtime SLAs start to go into effect on the downtime of connectors. They also do not include any downtime from their cloud provider.
- Deployment options: While Fivetran claims private cloud as an option, it has some serious limitations. Its private cloud deployment requires installation work and only supports 8 sources and 5 destinations. There is also a self-hosted option for HVR only.
- Support: Customers also complain about Fivetran support being slow to respond. Combined with reliability issues, this can lead to a substantial amount of data engineering time being lost to troubleshooting and administration.
- DataOps: Fivetran does not provide much control or transparency into what they do with data and schema. They alter field names and change data structures and do not allow you to rename columns. This can make it harder to migrate to other technologies. Fivetran also doesn’t always bring in all the data depending on the data structure, and does not explain why.
- Roadmap: Customers frequently comment Fivetran does not reveal much of a future direction or roadmap compared to the others in this comparison, and do not adequately address many of the above points.
Fivetran Pricing
Fivetran's pricing is based on monthly active rows (MAR). This can be very unpredictable because MARs are based on Fivetran’s internal representation of data, not yours. Any non-relational or nested data gets turned into highly normalized rows that raise costs.
Lower latency is also very expensive. To reduce latency from 1 hour to 15 minutes can cost you 33-50% more (1.5x) per million MAR, and 100% (2x) or more to reduce latency to 1 minute, which is rarely deployed. Some connectors require all data to be extracted each time, which also becomes more expensive as you lower latency and increase the number of extracts.
Even then, you still have the latency of the data warehouse load and transformations. The additional costs of frequent ingestions and transformations in the data warehouse can also be expensive and take time. Companies often keep latency high to save money.
While a small deployment (2M MARs/month) can cost $700-$2667, 10M MARs/month get you into $10K a month. It is not unheard of for Fivetran costs to reach 6 digits annually, especially with certain high-cost connectors that end up having many more MARs.
For those looking for Fivetran alternatives, it's worth considering solutions that offer lower costs, real-time streaming, or more flexibility in schema control.
Rivery
Rivery was founded in 2019. Since then it has grown to 100 people and 350+ customers. It’s a multi-tenant public cloud SaaS ELT platform. It has some ETL features, including inline Python transforms and reverse ETL. It supports workflows and can also load multiple destinations.
But Rivery is also similar to batch ELT. There are a few cases where Rivery is real-time at the source, such as with CDC, which is its own implementation. But even in that case it ends up being batch because it extracts to files and uses Kafka for file streaming to destinations which are then loaded in minimum intervals of 60, 15, and 5 minutes for the starter, professional, and enterprise plans.
If you’re looking for some ETL features and are OK with a public cloud-only option, Rivery is an option. It is less expensive than many ETL vendors, and also less expensive than Fivetran. But its pricing is medium-high for an ELT vendor.
Rivery's future offerings, policies, and pricing may be uncertain as they undergo an acquisition with Boomi.
Pros
- Modern data pipelines: Rivery is the one other modern data pipeline platform in this comparison along with Estuary.
- Transforms: You have an option of running Python (ETL) or SQL (ELT). You do need to make sure you use destination-specific SQL.
- Orchestration: Rivery lets you build workflows graphically.
- Reverse ETL: Rivery also supports reverse ETL.
- Load options: Rivery supports soft deletes (append only) and several update-in-place options including switch-merge (to merge updates from an existing table and switch), delete-merge (to delete older versions of rows), and a regular merge.
- Costs: Rivery is lower cost compared to other ETL vendors and Fivetran, though it is still higher than several ELT vendors.
Cons
- Batch only: While Rivery does extract from its CDC sources in real-time, which is the best approach, it does not support messaging sources or destinations, and only loads destinations in minimum intervals of 60 (Starter), 15 (Professional), or 5 (Enterprise) minutes.
- Data warehouse focus: While Rivery supports Postgres, Azure SQL, email, cloud storage, and a few other non data warehouse destinations, Rivery’s focus is data warehousing. It doesn’t support the other use cases as well.
- Public SaaS: Rivery is public cloud only. There is no private cloud or self-hosted option.
- Limited schema evolution: Rivery had good schema evolution support for its database sources. But the vast majority of its connectors are API-based, and those do not have good schema evolution support.
Rivery Pricing
Rivery charges per credit, which is $0.75 for Starter, $1.25 for Professional, and negotiated for Enterprise. You pay 1 credit per 100MB of moved data from databases, and 1 credit per API call. There is no charge for connectors. If you have low data volumes this will work well. But by the time you’re moving 20GB per month it starts to get more expensive than some others.
Estuary Flow
Estuary was founded in 2019. But the core technology, the Gazette open source project, has been evolving for a decade within the Ad Tech space, which is where many other real-time data technologies have started.
Estuary Flow is the only real-time and ETL data pipeline vendor in this comparison. There are some other ETL and real-time vendors in the honorable mention section, but those are not as viable a replacement for Fivetran. Estuary Flow is also a great option for batch sources and targets.
Where Estuary Flow really shines is in any combination of change data capture (CDC), real-time and batch ETL or ELT, and loading multiple destinations with the same pipeline. Estuary Flow currently is the only vendor to offer a private cloud deployment, which is the combination of a dedicated data plane deployed in a private customer account that is managed as SaaS by a shared control plane. It combines the security and dedicated compute of on-prem with the simplicity of SaaS.
CDC works by reading record changes written to the write-ahead log (WAL) that records each record change exactly once as part of each database transaction. It is the easiest, lowest latency, and lowest-load for extracting all changes, including deletes, which otherwise are not captured by default from sources. Unfortunately ELT vendors like Airbyte, Fivetran, Meltano, and Hevo all rely on batch mode for CDC. This puts a load on a CDC source by requiring the write-ahead log to hold onto older data. This is not the intended use of CDC and can put a source in distress, or lead to failures.
Estuary Flow has a unique architecture where it streams and stores streaming or batch data as collections of data, which are transactionally guaranteed to deliver exactly once from each source to the target. With CDC it means any (record) change is immediately captured once for multiple targets or later use. Estuary Flow uses collections for transactional guarantees and for later backfilling, restreaming, transforms, or other compute. The result is the lowest load and latency for any source, and the ability to reuse the same data for multiple real-time or batch targets across analytics, apps, and AI, or for other workloads such as stream processing, or monitoring and alerting.
Estuary Flow also has broad packaged and custom connectivity, making it one of the top ETL tools. It has 150+ native connectors that are built for low latency and/or scale. While this number may seem low, these are high-quality, standardized connectors. In addition, Estuary is the only vendor to support Airbyte, Meltano, and Stitch connectors, which easily adds 500+ more connectors. Getting official support for the connector is a quick “request-and-test” with Estuary to make sure it supports the use case in production. Most of these connectors are not as scalable as Estuary-native, Fivetran, or some ETL connectors, so it’s important to confirm they will work for you. Flow’s support for TypeScript and SQL transformations also enables ETL.
Pros
- Modern data pipeline: Estuary Flow has the best support for schema drift, evolution, and automation, as well as modern DataOps.
- Modern transforms: Flow is also both low-code and code-friendly with support for SQL and TypeScript (with Python on the way) for ETL, and dbt for ELT.
- Lowest latency: Several ETL vendors support low latency. But of these Estuary can achieve the lowest, with sub-100ms latency. ELT vendors generally are batch only.
- High scale: Unlike most ELT vendors, leading ETL vendors do scale. Estuary is proven to scale with one production pipeline moving 7GB+/sec at sub-second latency.
- Most efficient: Estuary alone has the fastest and most efficient CDC connectors. It is also the only vendor to enable exactly-and-only-once capture, which puts the least load on a system, especially when you’re supporting multiple destinations including a data warehouse, high performance analytics database, and AI engine or vector database.
- Deployment options: Of the ETL and ELT vendors, Estuary is currently the only vendor to offer open source, private cloud, and public multi-tenant SaaS.
- Reliability: Estuary’s exactly-once transactional delivery and durable stream storage makes it very reliable.
- Ease of use: Estuary is one of the easiest to use tools. Most customers are able to get their first pipelines running in hours and generally improve productivity 4x over time.
- Lowest cost: For data at any volume, Estuary is the clear low-cost winner in this evaluation. Rivery is second.
- Great support: Customers consistently cite great support as one of the reasons for adopting Estuary.
Cons
- On premises connectors: Estuary has 150+ native connectors and supports 500+ Airbyte, Meltano, and Stitch open source connectors. But if you need on-premises app or data warehouse connectivity, make sure you have all the connectivity you need.
- Graphical ETL: Estuary has been more focused on SQL and dbt than graphical transformations. While it does infer data types and convert between sources and targets, there is currently no graphical transformation UI.
Estuary Flow Pricing
Of the various ELT and ETL vendors, Estuary is the lowest total cost option. Estuary only charges $0.50 per GB of data moved from each source or to each target, and $100 per connector per month. Rivery, the next lowest cost option, is the only other vendor that publishes pricing of 1 RPU per 100MB, which is $7.50 to $12.50 per GB depending on the plan you choose. Estuary becomes the lowest cost option by the time you reach the 10s of GB/month. By the time you reach 1TB a month Estuary is 10x lower cost than the rest.
How to choose the best option
For the most part, if you are interested in a cloud option, and the connectivity options exist, you may choose to evaluate Estuary.
Modern data pipeline: Estuary has the broadest support for schema evolution and modern DataOps.
Lowest latency: If low latency matters, Estuary will be the best option, especially at scale.
Highest data engineering productivity: Estuary is among the easiest to use, on par with the best ELT vendors. But it also has delivered up to 5x greater productivity than the alternatives.
Connectivity: If you're more concerned about cloud services, Estuary or another modern ELT vendor may be your best option. If you need more on-premises connectivity, you might consider more traditional ETL vendors.
Lowest cost: Estuary is the clear low-cost winner for medium and larger deployments.
Streaming support: Estuary has a modern approach to CDC that is built for reliability and scale, and great Kafka support as well. It's real-time CDC is arguably the best of all the options here. Some ETL vendors like Informatica and Talend also have real-time CDC. ELT-only vendors only support batch CDC.
Ultimately the best approach for evaluating your options is to identify your future and current needs for connectivity, key data integration features, and performance, scalability, reliability, and security needs, and use this information to a good short-term and long-term solution for you.
Related comparisons to Fivetran
Related comparisons to Rivery
Getting started with Estuary
Free account
Getting started with Estuary is simple. Sign up for a free account.
Sign upDocs
Make sure you read through the documentation, especially the get started section.
Learn moreCommunity
I highly recommend you also join the Slack community. It's the easiest way to get support while you're getting started.
Join Slack CommunityEstuary 101
I highly recommend you also join the Slack community. It's the easiest way to get support while you're getting started.
Watch
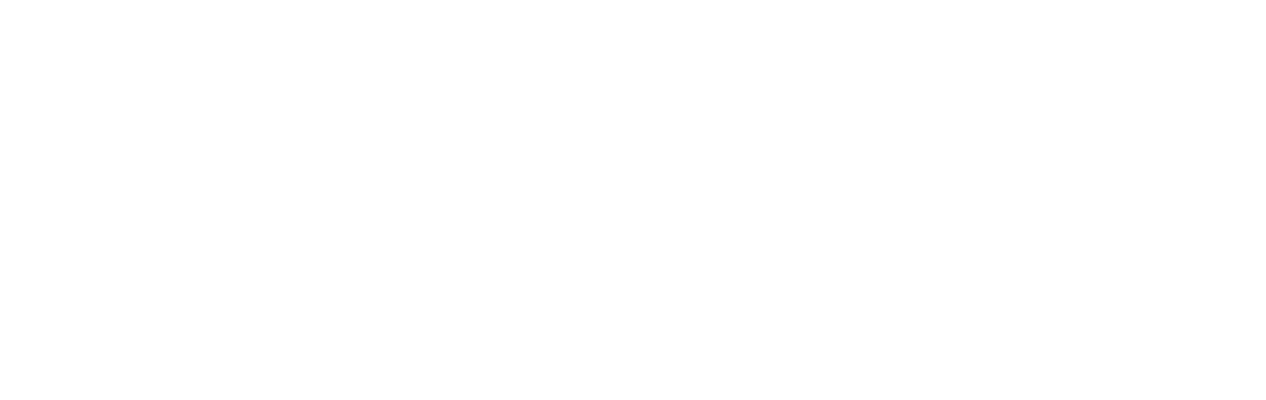