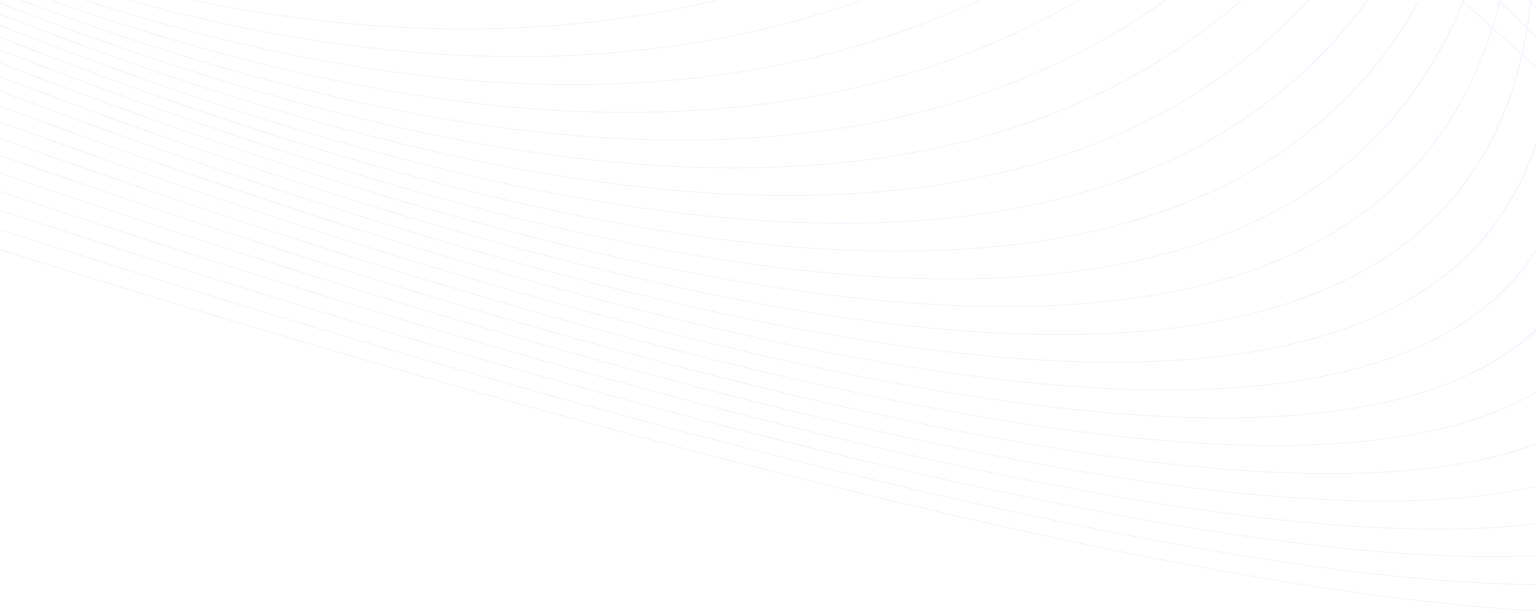
Data flows through every aspect of our lives, from the products we buy online to the services we use daily. But here's the thing – data is only as valuable as it is accurate and reliable. If you base your decisions on unclean, inaccurate data, your business operations can suffer serious consequences. This is where data cleansing clears away the fog surrounding the data.
From financial institutions protecting sensitive information to businesses fine-tuning their marketing strategies, data cleansing shores up the core of many different operations. It might sound technical, but it's a practice that every organization, big or small, should understand and embrace.
If you happen to be thinking about giving data cleansing a go, well, you’re in the right place. In this guide, we'll explore the core concepts of data cleansing, what tools to use, why it's important, and give you practical insights on how to effectively cleanse your data.
What Is Data Cleansing?
Data cleansing, also known as data cleaning or data scrubbing, is the methodical correction or removal of errors, inconsistencies, and inaccuracies present within datasets. The main objective of data cleansing is to enhance the quality and reliability of data and make it suitable for various applications like analytics, reporting, and decision-making.
Errors in datasets can take different forms, including typographical errors (typos!), missing values, duplicate entries, inconsistent formatting, and outliers. These errors can be because of reasons like manual data entry, system glitches, data integration, or data migration processes. Without proper data cleansing, these inaccuracies can compromise the data integrity and cause incorrect conclusions and potentially costly mistakes.
Why Do You Need To Perform Data Cleansing?
Data cleansing is an important task in data management. But why do you need to perform it? Well, there are several compelling reasons and we're about to discuss them in detail.
Improve Data Accuracy
First and foremost, data cleansing is all about improving data accuracy. Imagine you're making critical business decisions based on data that's riddled with errors and inconsistencies. That's a recipe for disaster, right? Clean data ensures that your information is reliable and trustworthy.
When you perform data cleansing, you're weeding out inaccuracies, errors, and outliers that may have crept into your dataset. These inaccuracies could be because of human errors during data entry or inconsistencies in the data sources. Through data cleaning, you're improving its quality which in turn boosts the accuracy of any analysis or reporting you do.
Accurate data results in more informed decisions, better customer service, and improved operational efficiency. But how can you go about eliminating errors and reducing consistency from the get-go?
Fortunately, powerful SaaS tools like Estuary Flow can help you to improve your data’s accuracy. For instance, Flow can capture data from a variety of sources in real time which means that your data is always current and reliable.
This is important because data can change quickly and if you are using outdated data, your analysis or reporting will be inaccurate. But, we’ll talk about Estuary Flow in more detail a bit further along in this article. Before getting ahead of ourselves (!), let’s look at some more reasons for performing data cleansing.
Eliminate Duplicates
Duplicate data can be a real problem. Imagine you have 2 entries for the same customer in your database. One says they live in New York and the other says Los Angeles. Which one is correct? Without data cleansing, you might end up sending marketing materials to both addresses, wasting resources and annoying your customers in the process.
When you eliminate duplicate data through data cleansing, you streamline your records and ensure that each piece of information is unique and accurate. This not only saves you time and money but also enhances your customer relationships. Nobody likes receiving multiple copies of the same email, right?
Enhance Data Completeness
Incomplete data won't give you the whole picture. When you cleanse data, you make sure that all necessary information is present and accounted for.
Imagine you're a business trying to understand your customer demographics. If your customer data lacks crucial details like age, location, or contact information, you're working with a partial view. This can make it tough for you to customize your marketing strategies or provide personalized services.
Data cleansing fills the gaps to enhance data completeness. You're not just removing irrelevant data but also making sure that important information is available. This comprehensive data is like gold for data scientists and analysts who rely on complete datasets to get meaningful conclusions and make informed decisions.
Support Better Decision-Making
Data-driven decision-making is a hot topic these days, and for good reason. But, for it to work effectively, the data you base your decisions on must be reliable and accurate. Data cleansing plays a major role in ensuring this.
When your data is clean, accurate, and complete, it becomes a trustworthy foundation for decision-making. Business leaders, data scientists, and analysts can confidently rely on this data to identify trends, spot opportunities, and mitigate risks.
On the other hand, relying on messy, inconsistent, or incomplete data can cause misguided decisions, potentially resulting in financial losses or missed opportunities. So data cleansing isn't just about cleaning up data; it's about empowering better decision-making across the board.
Reduce Operational Inefficiencies
Operational inefficiencies can be a real drain on resources and productivity. Imagine having to deal with duplicate records, inconsistent data formats, and irrelevant information. It's a nightmare for data scientists and operational teams alike.
Data cleansing can help reduce these operational inefficiencies significantly. When you remove duplicates, standardize data, and get rid of irrelevant data, you simplify data management processes. As a result, operations become much more streamlined, and productivity increases.
For example, when you're trying to generate reports or conduct data analysis, having clean and consistent data saves you hours of manual effort that would otherwise be spent on data correction and reconciliation.
Ensure Data Consistency
Consistency is key in the data world. If your data isn't consistent, it can cause confusion and misinterpretation. Data cleansing plays a vital role in ensuring that your data is consistent across the board.
For example, let's say you're analyzing sales figures and one part of your dataset uses "USD" for currency, while another uses "U.S. Dollars.” These variations might seem minor but they can cause issues when you're trying to aggregate or compare data.
Through data cleansing, you can standardize your data and make sure that all units of measurement, date formats, and other attributes adhere to a consistent structure. This consistency simplifies data transformation and manipulation, making it easier to draw meaningful insights from your dataset.
Enhance Customer Satisfaction
Customer data is what helps you personalize your services, target your marketing efforts, and build lasting relationships. But what happens when your customer data is riddled with errors, duplicates, or inconsistencies? Customer satisfaction takes a hit.
Imagine receiving a promotional email that addresses you as "John Doe" one day and "Jane Smith" the next. It doesn't exactly make you feel valued. Data cleansing ensures that your customer data is accurate and up-to-date to enhance customer satisfaction.
When you use clean data to personalize communications and tailor services, you show your customers that you know and care about them. This boosts their satisfaction, loyalty, and trust in your brand.
Comply With Data Regulations
Data privacy regulations have become a major part of the modern business landscape. Non-compliance can cause hefty fines and a damaged reputation. Here's where data cleansing comes into play.
When you clean your data and make sure it's accurate, current, and relevant, you're moving in the right direction to meet these data rules and regulations. It helps you avoid mishandling customer data or storing information you shouldn't, reducing the risk of legal troubles.
Data cleansing also supports the "right to be forgotten" – a key aspect of data regulations. When you clean your data, you can easily identify and remove customer data upon request. This ensures compliance and helps maintain your reputation as a responsible data custodian.
Minimize Financial Risks
Data errors can be costly. If your financial records have structural errors or inconsistencies, it can cause financial discrepancies, losses, or even financial audits. Data cleansing helps minimize these financial risks.
Through the data cleansing process, you reduce the chances of financial mismanagement or misreporting. This can save your company significant sums of money and protect you from financial penalties or litigation.
Data cleansing provides you with a financial safety net to make sure that your records are trustworthy and reliable, thus minimizing the financial risks associated with erroneous data.
Enable Effective Data Analytics
Effective data analytics relies on clean, reliable data. If your dataset is filled with duplicates, inconsistencies, or irrelevant information, your analytics efforts can quickly become a frustrating exercise.
Data cleansing equals effective data analytics. When your data is clean, data scientists and analysts can confidently extract insights, identify patterns, and make data-driven recommendations.
Also, clean data helps in more accurate predictive modeling. You can foresee market trends, understand your customers inside out, and spot risks on the horizon – a major leg up in today’s data-driven world.
How Do You Perform Data Cleansing? A Detailed Look Into Data Cleaning Techniques
Data cleansing is a critical step in ensuring your data’s accuracy and reliability. While it may not be a difficult process, it does require a systematic approach to be truly effective. Let's break down the key components of this important data management practice.
Define Data Cleansing Goals
- Assessment: Start by understanding your data. What does it look like? What's its source? What are the common issues you're dealing with? Is it missing data, duplicates, or something else?
- Define Clear Objectives: Determine what you want to achieve with data cleansing. Are you aiming for accuracy, consistency, or compliance with certain standards?
- Set Priorities: Identify which data elements are critical and prioritize them for cleansing.
- Create Data Quality Rules: Establish rules and criteria for what constitutes "clean" data. For example, you might specify that all dates should be in a standard format.
Handle Missing Values
- Identify Missing Data: Use data profiling techniques or data cleansing tools to pinpoint where the missing data is. This could be in columns, rows, or even entire datasets.
- Determine Strategy: Decide how you want to handle missing data. Common strategies include:
- Removing rows or columns with a high percentage of missing values if they don't carry essential information.
- Imputing missing values using techniques like mean, median, or mode replacement.
- Using advanced methods like predictive modeling to fill in missing values when appropriate.
- Removing rows or columns with a high percentage of missing values if they don't carry essential information.
- Document Changes: Keep track of any changes made to the data, especially when imputing missing values, so you can explain your decisions later.
Remove Duplicates
- Detect Duplicate Entries: Use data cleaning tools or manual inspection to identify duplicate records. These can be records with identical values across specific columns or even partial matches.
- Select a Deduplication Method: Choose an appropriate method for dealing with duplicates:
- Simple removal: Delete the duplicate records, keeping only one.
- Aggregation: Merge duplicates by consolidating information.
- Flagging: Mark duplicates for manual review or further investigation.
- Simple removal: Delete the duplicate records, keeping only one.
- Perform Deduplication: Execute the chosen method systematically across your data to ensure consistency.
- Quality Assurance: Double-check your work to make sure you haven't accidentally deleted or modified legitimate data.
Standardize Data Formats
- Data Format Identification: Identify inconsistent data formats within your dataset. These can include date formats, currency symbols, units of measurement, and more.
- Define Standard Formats: Establish a set of standard formats that your data should adhere to. For example, all dates should be in YYYY-MM-DD format, or currency amounts should use a consistent currency symbol and number of decimal places.
- Automate Standardization: Use tools or scripts to automatically convert data into the standard formats you've defined. This helps ensure uniformity across your dataset.
- Manual Intervention: In cases where data format standardization cannot be automated because of complexity or uniqueness, perform manual adjustments while sticking to the established standards.
Correct Data Inaccuracies
- Identify Inaccurate Data: Pinpoint inaccuracies in your data. This could be anything from misspelled names to inconsistent date formats or incorrect values.
- Data Cleansing Tools: Employ data cleansing tools and techniques to automatically correct common inaccuracies. Some tools can standardize data formats, spell-check text, and even validate data against predefined rules.
- Manual Review: For more complex inaccuracies or those that can't be automated, perform a manual review. This can be cross-referencing data with external sources or consulting subject matter experts.
- Documentation: Keep detailed records of the corrections made so you have a clear audit trail of the changes.
Validate Data Integrity
- Data Validation Rules: Define validation rules to ensure that your data meets specific criteria. For instance, you might have rules to validate email addresses, phone numbers, or financial transactions.
- Automated Validation: Use data validation tools to automatically check data against these rules. The tools will flag any data that doesn't comply with the defined criteria.
- Manual Validation: In some cases, manual validation is necessary, especially when dealing with complex or unique data elements. Review data carefully to ensure its integrity.
- Error Handling: Develop a strategy for handling validation errors. You might choose to reject invalid data, send it for manual review, or apply specific transformations to bring it into compliance.
Transform & Format Data
- Data Transformation: Sometimes, data needs to be transformed to fit a specific format or structure. This can involve converting units of measurement, translating text into different languages, or aggregating data.
- Data Formatting: Standardize your data formatting to maintain consistency. This could include date formatting, capitalization rules, or numerical precision.
- Normalization: Normalize data to reduce redundancy and improve efficiency. For instance, you might split a combined "full name" field into separate "first name" and "last name" fields.
- Data Enrichment: Add relevant information from external sources to enhance your data. This can improve your data quality and context.
Conduct Outlier Detection
- Identify Outliers: Outliers are data points that deviate significantly from the majority of the data. Use statistical methods like z-scores or interquartile range (IQR) to identify them.
- Define Thresholds: Establish thresholds for what constitutes an outlier in your specific dataset. This might involve specifying a range of acceptable values or determining outliers based on domain knowledge.
- Flag or Correct Outliers: Decide how to handle outliers. You can flag them for further investigation, correct them if they result from errors, or remove them if they're extreme and unlikely to be valid data.
Perform Data Quality Checks
- Data Quality Metrics: Define specific data quality metrics that are relevant to your dataset. These metrics could include accuracy, completeness, consistency, and timeliness.
- Automated Checks: Use data quality tools to automate the measurement of these metrics. These tools can generate reports and alerts when data quality falls below acceptable levels.
- Manual Validation: Some aspects of data quality, like context and relevance, may require manual validation. Experts can assess the data against business rules and standards.
- Documentation: Maintain comprehensive documentation of your data quality checks, including the metrics measured, thresholds applied, and actions taken to address issues.
Test The Cleansed Data
- Test Scenarios: Develop test scenarios that mimic real-world situations where your cleansed data will be used. This could involve running queries, conducting analyses, or generating reports.
- Compare Results: Execute these test scenarios using both the original, uncleaned data and the newly cleansed data. Compare the results to identify discrepancies.
- Validation against Business Rules: Ensure that the cleansed data follows established business rules, standards, and requirements.
- Iterative Process: If discrepancies or issues arise during testing, revisit earlier steps in the data cleansing process to make necessary corrections. Testing should be iterative until the data meets your defined criteria.
How Does Estuary Flow Help With Data Cleansing?
Estuary Flow is our user-friendly, no-code DataOps platform that effortlessly handles real-time data ingestion, transformation, and replication. It's designed to tackle any failures and offers exactly-once semantics for reliable and seamless data processing.
Estuary Flow comes with built-in data cleansing and transformation features that make cleaning and enriching your extracted data easy. These features make it a cinch to get your data ready for analysis or seamless integration with other systems.
Let’s look at how Estuary Flow can drive a clean data culture in your organization.
Efficient Data Capture
Estuary Flow gathers data from various sources, like databases and applications. Through Change Data Capture (CDC), Estuary ensures that it captures only the most recent and up-to-date data. No more outdated data and duplicates. Also, built-in schema validation ensures only clean data enters the pipeline.
Dynamic Data Manipulation
Once the data enters the system, Estuary Flow lets you tailor it to your specific needs. With the flexibility of streaming SQL and JavaScript, you can effortlessly shape your data as it flows.
Comprehensive Quality Assurance
Estuary incorporates an integrated testing framework. This advanced testing system guarantees that your data pipelines remain error-free and dependable. Should any irregularities arise, Estuary promptly alerts you to prevent corrupt data from infiltrating your destination system.
Sustaining Data Integrity
Estuary Flow not only ensures initial data cleanliness but also maintains it over time. It creates low-latency views of your data that are consistently refreshed. This approach ensures that your data remains uniform across various systems, maintaining its reliability.
Data Integration
Estuary serves as the architect of data convergence. It seamlessly integrates data from different sources to give you a comprehensive view. This guarantees that you have high-quality information so that you can make well-informed decisions and deliver superior services.
Conclusion
Data cleansing saves you time, money, and headaches. So, approach it systematically, use the right tools, and make data quality an ongoing commitment – not just a one-time task. Embrace it as an opportunity to dig up the information buried within your data to make better decisions.
Estuary Flow is a real-time data movement and transformation platform that can help you cleanse your data quickly and easily. It offers a wide range of built-in transformations that can be used to cleanse data. Estuary is also highly scalable so it can handle even the largest datasets. With its cloud-based architecture, you can get started quickly and easily, without having to worry about managing any infrastructure.
Sign up for Flow to start for free or contact our team to discuss your specific needs.
Frequently Asked Questions About Data Cleansing
If you still have questions about the ins and outs of data cleansing, you're about to find answers. Let’s get started.
What are the characteristics of quality data?
Here are 10 major characteristics of high-quality data:
- Accuracy: Data should be free from errors and discrepancies.
- Completeness: It should include all relevant information.
- Consistency: Data should be uniform and follow standardized formats.
- Timeliness: Data should be up-to-date and relevant to the task.
- Relevance: It should be directly related to the purpose or analysis.
- Reliability: Data should be trustworthy and consistent over time.
- Precision: Information should be detailed and granular when necessary.
- Accessibility: Data should be easily accessible to authorized users.
- Security: Measures should protect data from unauthorized access or loss.
- Validity: Information should conform to defined rules and criteria.
What is an example of data cleaning?
Imagine you have a dataset of customer addresses and you notice that some entries have inconsistent formats, like "123 Main St." versus "123 Main Street." Data cleaning in this case involves standardizing the address format by converting all variations to a consistent style, say, "123 Main Street".
It might also include removing duplicates, correcting typos, and filling in missing postal codes. Data cleaning ensures your dataset is tidy, accurate, and ready for analysis, preventing errors that could arise from messy data.
What is a data cleansing tool?
A data cleansing tool is software designed to help automate the process of cleaning and improving the data quality. These tools identify and rectify errors, inconsistencies, and inaccuracies within datasets. They can perform tasks like removing duplicates, standardizing formats, correcting spelling mistakes, and validating data against predefined rules.
What are some of the best data cleansing tools?
There are several popular data cleansing tools that you can use for data cleaning, but some of the best tools include:
- OpenRefine
- TIBCO Clarity
- Informatica Cloud Data Quality
- WinPure Clean & Match
- DemandTools
- Alteryx (Trifacta Wrangler)
- IBM Infosphere Quality Stage
How do I clean data in SQL?
Cleaning data in SQL involves using SQL queries to identify and rectify issues within your dataset. Here are the common techniques:
- Remove Duplicate Rows: Use the DISTINCT keyword or GROUP BY clause to eliminate duplicate rows.
- Correct Data Types: Use CAST or CONVERT functions to change data types when needed, e.g., from string to date.
- Handling Missing Values: Use IS NULL or IS NOT NULL to identify and handle missing data, either by filling them or excluding rows.
- Standardize Text Data: Use functions like UPPER, LOWER, and TRIM to standardize text formats.
- Data Transformation: Use UPDATE statements to modify data, like correcting spelling mistakes.
- Filtering Outliers: Use WHERE clauses to filter out values that are outliers or erroneous.
- Validation Rules: Apply constraints to enforce data integrity rules.
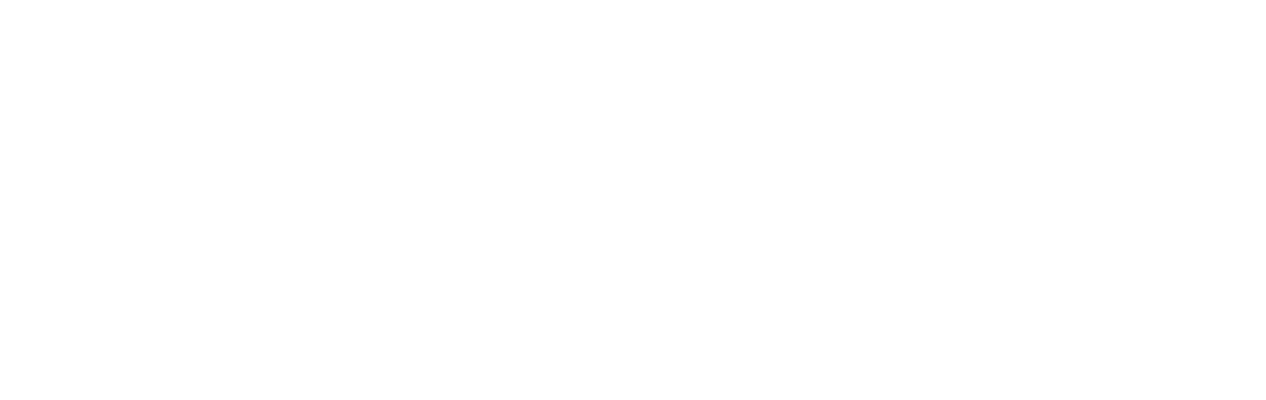
About the author
With over 15 years in data engineering, a seasoned expert in driving growth for early-stage data companies, focusing on strategies that attract customers and users. Extensive writing provides insights to help companies scale efficiently and effectively in an evolving data landscape.
Popular Articles
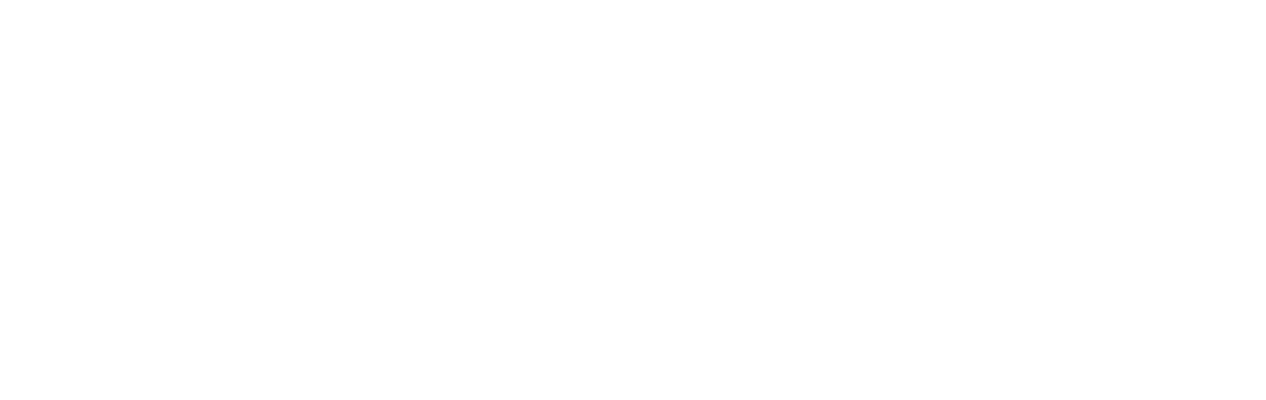