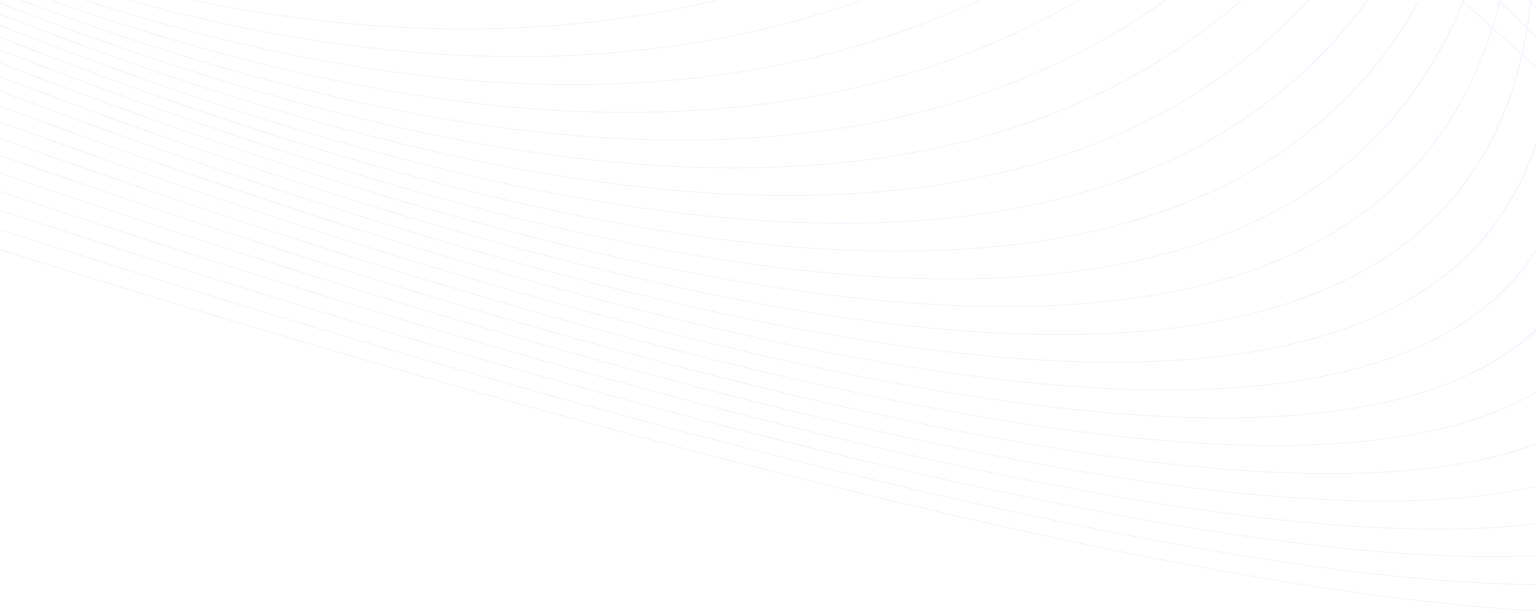
Are you frustrated with unpredictable costs when syncing data across your systems? For many organizations, Fivetran's pricing can be a double-edged sword. While it simplifies data integration, its Monthly Active Row (MAR) pricing model often leads to difficulty managing or predicting cost escalations.
Struggling with unpredictable data integration costs? Try Estuary Flow for free and get transparent, cost-effective pricing for real-time and batch data pipelines.
Data integration is the backbone of modern analytics, and selecting the right tool is critical to your budget and operations. Whether you’re processing real-time streams, managing Change Data Capture (CDC) workloads, or syncing large datasets, understanding how pricing works can save your organization from significant hidden costs.
In this blog, we’ll take a deep dive into Fivetran’s pricing model, breaking down how MAR-based pricing works, why it can become expensive for high-volume workloads, and how alternative solutions like Estuary Flow offer a more transparent and cost-efficient model. If you’re evaluating Fivetran or looking for a more innovative alternative, this article will help you make an informed decision.
Let’s start by understanding the intricacies of Fivetran’s pricing and the challenges it poses for scaling your data infrastructure.
Suggested Read: What is Fivetran?
Fivetran Pricing Explained: How MAR Pricing Works & Hidden Costs
Fivetran’s Monthly Active Row (MAR) pricing model is designed to charge users based on the number of rows in their dataset that are updated or added within a month. While this sounds simple, several factors can cause costs to escalate rapidly, especially for organizations with high-volume or complex data.
2025 Fivetran Pricing Update
In 2025, Fivetran announced a major shift in its pricing strategy, moving from account-based Monthly Active Row (MAR) discounts to connector-level discounts. While this might seem minor at first glance, it has significant implications for organizations using multiple connectors.
What’s Changing?
Previously, Fivetran offered MAR-based discounts at the account level, meaning businesses with high data volumes across various connectors could benefit from aggregated discounts. Now, with connector-level discounts, each connector’s usage is evaluated independently, and discounts are applied separately to each connector.
Why This Matters
For companies relying on multiple connectors—common in complex data environments—this change can result in substantial cost increases. Here’s why:
- Loss of Aggregated Discounts: Businesses can no longer leverage high-volume discounts across their entire account. Instead, they’re required to reach discount thresholds on each individual connector, which is often unrealistic unless data volumes are extremely high on every connector.
- Higher Costs for Multi-Connector Setups: Companies using numerous connectors to integrate data from different sources (e.g., Salesforce, HubSpot, NetSuite, etc.) may see their costs skyrocket as each connector is priced independently.
- Unpredictable Budgeting: The shift adds another layer of complexity to budgeting and cost management. Predicting costs across multiple connectors with varying data volumes becomes increasingly difficult.
Real-World Impact
Imagine a business using five connectors, each contributing to a total MAR that previously qualified for a significant discount. Under the new pricing model, the business will face higher costs unless each of those connectors individually meets the discount threshold. This could lead to unexpected budget overruns, particularly for organizations scaling their data infrastructure.
How Fivetran’s Monthly Active Row (MAR) Pricing Works
MAR pricing charges are based on unique rows that are modified or added each month. But this model introduces several complexities:
However, the MAR pricing model introduces several layers of complexity:
- Normalized Data Increases MAR Counts: Fivetran processes and normalizes data from the source, often splitting a single record into multiple rows. This process inflates the MAR count, particularly for semi-structured data such as JSON or nested formats.
- Frequent Updates Drive Costs: Any changes to the data, even minor updates to a single field, count toward the MAR total, further increasing your bill.
Key Challenges of Fivetran’s Pricing Model
1. Unpredictable Costs
- Complex data structures or frequent updates can lead to unexpected cost spikes.
- For example, syncing 1 million rows of nested JSON could result in 10+ million MARs after normalization.
Because of these factors, estimating future costs with a MAR-pricing model is a near-impossible task.
2. High Costs for Streaming and Real-Time Workloads
- Each unique event in real-time pipelines (e.g., data coming from Kafka, Kinesis, or some other event-based source) contributes to MAR counts.
- This makes streaming workloads prohibitively expensive for businesses handling continuous data streams.
3. Scaling Challenges with High-Volume Data
- Organizations processing large datasets or Change Data Capture (CDC) workloads face escalating costs as data volume grows.
- Fivetran’s High Volume Agent (HVA), designed to handle CDC workloads with over 100 million row changes per month, comes at an additional cost and requires installing custom software on your source database—introducing operational complexity and potential security risks.
4. Penalty for Semi-Structured or Nested Data
- Businesses using JSON, XML, or similar formats see higher MAR counts due to splitting nested fields into multiple rows.
Real-World Cost Implications of Fivetran
Let’s look at some real-world examples of Fivetran’s pricing in action:
- Small Deployment: A business syncing 2 million MARs per month can expect to pay anywhere between $700 to $2,667/month, depending on the complexity of the connector and data structure.
- High-Volume Use Case: For organizations processing 10 million MARs per month, monthly costs often exceed $10,000, with some businesses reporting six-figure annual bills for larger deployments.
- Streaming Workloads: Companies relying on real-time data pipelines (e.g., clickstream data or application events) often see exponential cost increases. Every unique event in a stream adds to the MAR count, leading to bills that grow unpredictably with data volume.
A HubSpot Example: Cascading MAR Across Entities
HubSpot's nested data structure means that updates to one entity often cascade, triggering updates across related entities. This cascading behavior amplifies the Monthly Active Rows (MAR) count, as interactions, updates, and logs ripple through the system. Here’s how MAR cascades across entities in a growing business:
- Contacts: Each interaction, lifecycle stage update, or property change triggers a row update.
- Deals: Pipeline stage transitions, value adjustments, or added notes generate row churn.
- Marketing Emails: Clicks, opens, and bounces create activity logs.
- Custom Properties: Updates to custom fields across entities contribute heavily to row volume.
With Fivetran’s MAR pricing, businesses are penalized for data activity, not data value.
Hidden Costs in Fivetran’s Pricing
Despite its simplicity, Fivetran’s pricing often leads to unexpected charges, including:
- Normalization Inflation: Semi-structured data can balloon MAR counts after Fivetran normalizes it.
- Schema Changes: Even minor schema updates in source data can unexpectedly increase costs.
- Unused Connectors: Some users report paying for connectors that are only occasionally used but still count toward overall MARs.
These costs are often hard to predict, making budgeting for Fivetran challenging, especially for growing organizations.
For more insights on the real-world impact of Fivetran’s pricing, check out this Reddit discussion. Users in the thread share their experiences with Fivetran’s unpredictability, highlighting the challenges many face when syncing large or complex data sources.
💡 Looking for a cost-effective alternative? Check out this list of top Fivetran alternatives to explore solutions that offer better pricing models and flexibility for your data pipelines.
Estuary Flow vs. Fivetran Pricing: A Transparent Alternative
Estuary Flow offers a straightforward and transparent pricing model that empowers businesses to scale data integration without worrying about hidden fees or unpredictable costs. Unlike Fivetran’s Monthly Active Row (MAR) pricing, Estuary Flow’s pricing is designed to align with your actual data consumption, making it easier to predict and manage your costs.
Key Features of Estuary Flow Pricing
- Volume-Based Pricing: Estuary Flow charges based on data volume (in TB) rather than per row, making it easier to estimate your monthly expenses. This model is particularly advantageous for businesses managing large datasets or high-frequency changes, as costs remain predictable regardless of the number of rows or schema complexity.
- No Hidden Fees: Estuary Flow eliminates the need for additional tools or custom agents, unlike Fivetran’s High Volume Agent (HVA) for CDC workloads, which requires extra costs and operational complexity.
- Cost-Efficient for Real-Time Data: Estuary Flow’s pricing is particularly cost-effective for real-time data pipelines, including streaming workloads from sources like Kafka or Kinesis. Since Estuary Flow charges by data volume, you won’t face inflated costs due to unique events in a stream, unlike Fivetran’s MAR-based charges.
- Built for Scalability: Whether you’re syncing small, infrequent data updates or large-scale CDC workloads, Estuary Flow provides a flexible and scalable solution that grows with your business needs.
Using Estuary Flow’s pricing calculator, a business moving 250 GB of data per month with 2 connectors would pay $450/month. In comparison, Fivetran would charge $10,199/month for the same data volume. This cost efficiency is a game-changer for organizations handling large datasets or streaming workloads.
Use Estuary Flow's pricing calculator to easily estimate your monthly costs based on your requirements, offering you control and predictability without the complexity of hidden fees.
Final Thoughts
Fivetran’s MAR pricing may work for small-scale, low-frequency use cases, but its limitations become apparent as data complexity and volume grow. Estuary Flow offers a transparent, scalable alternative designed to meet the demands of modern data integration.
Ready to take control of your data integration costs? Try Estuary Flow for Free and experience seamless, cost-efficient data pipelines.
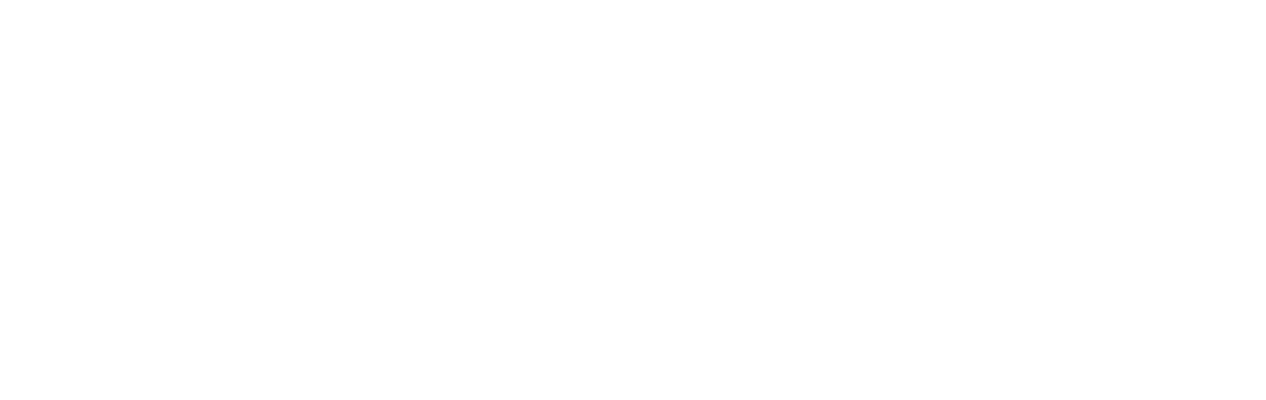
About the author
Dani is a data professional with a rich background in data engineering and real-time data platforms. At Estuary, Daniel focuses on promoting cutting-edge streaming solutions, helping to bridge the gap between technical innovation and developer adoption. With deep expertise in cloud-native and streaming technologies, Dani has successfully supported startups and enterprises in building robust data solutions.
Popular Articles
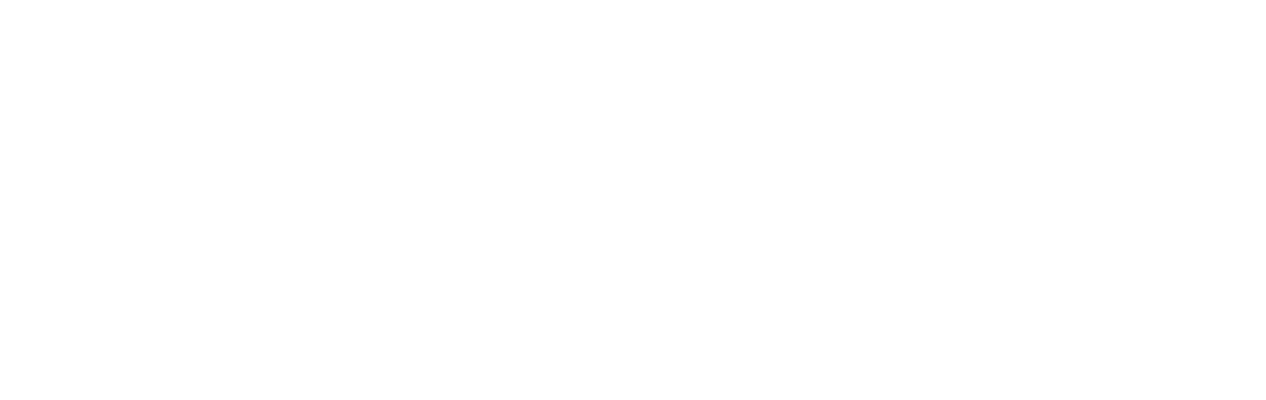