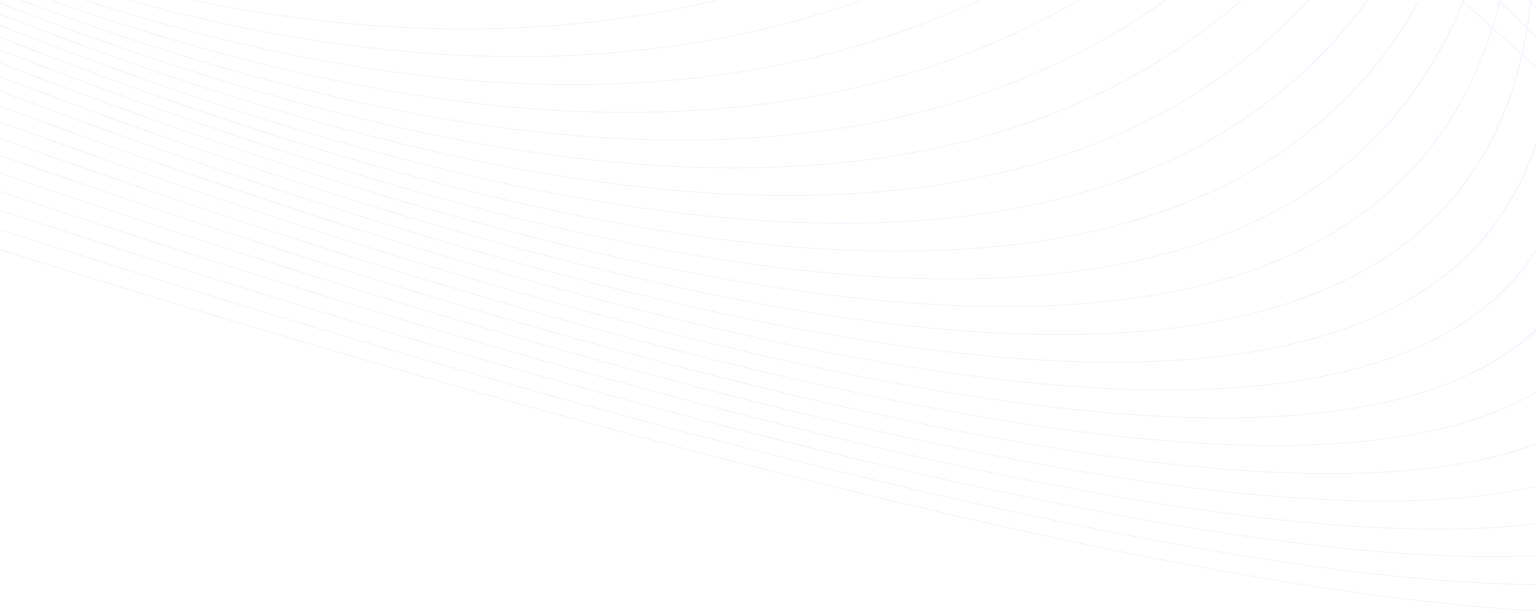
Ever wait around for a shipment that was supposed to arrive “around noon,” only for it to show up at 4:37 PM? Yeah, same. That kind of guesswork used to be par for the course in freight. But now? It’s outdated. Carriers still relying on static ETAs (Estimated Time of Arrival) are flying blind in a world that demands precision and real-time freight tracking.
These days, the pressure’s on to deliver accurate, real-time freight ETA predictions, and honestly, it’s not just a “nice-to-have” anymore. It's become something customers expect. Behind the scenes, machine learning in logistics is powering that shift—and the most innovative freight engineers are using it to get a serious leg up.
This article explores how predictive ETAs powered by real-time data and machine learning are transforming freight logistics—improving accuracy, reducing delays, and driving smarter, adaptive transportation systems.
What’s Wrong With Static ETAs Anyway?
On paper, static ETAs seem fine. The system looks at pickup time, estimates the drive, maybe adds a bit for traffic, and spits out a number. Done, right?
Well… no.
Freight transportation doesn’t exist in a vacuum. Stuff happens. A driver might hit their HOS (Hours of Service) limit halfway through. Traffic turns gnarly just past the state line. A facility holds a truck longer than usual. Snow starts falling. Static ETA systems just don’t see that stuff coming. They weren’t built for it.
Predictive ETAs systems, on the other hand, are trained to expect the unexpected. They learn from previous delays. They adjust. And they get smarter with every trip.
So, What Actually Goes Into a Predictive ETA System?
Source: uber.com
Let’s break it down without getting too nerdy.
A solid freight ETA prediction system usually includes the following:
- A data ingestion layer – This pulls in info from GPS, vehicle sensors, TMS data, traffic, and weather APIs, you name it.
- Feature extraction logic – Translates raw data into signals that a model can learn from.
- The model itself – Could be a simple regressor or a deep learning model, depending on complexity.
- Real-time inference tools – This is so the system can make predictions on demand.
- A feedback loop – Where the system learns when it gets something wrong so it can improve.
Nothing magical here—just a lot of moving parts working together to guess better than humans can.
The Data That Makes Predictive Freight ETAs Work
A model’s only as good as what you feed it. And in freight logistics, you’ve got all kinds of signals to work with:
- Live vehicle data – Speed, location, acceleration patterns.
- External stuff – Weather, traffic flow, road closures.
- Historical trends – Previous runs, average wait times at docks.
- Ops info – HOS logs, appointment schedules, even driver habits.
It’s a soup. And cleaning it up is half the battle.
Engineering Features That Actually Matter
Raw data is one thing. But you’ve gotta build it into something useful. That’s where feature engineering comes in.
Some of the heavy hitters?
- Time-of-day context – Because 8 AM in Dallas traffic ≠ , 8 AM in Montana.
- Weather-based speed changes – Some drivers slow down more than others in the rain.
- Live vs. historical comparisons – To flag when a route’s moving slower than usual.
- Driver or fleet-specific behavior – Useful when predicting how a certain carrier typically performs.
And yep, that’s all stuff you can feed into a model. Sometimes, it’s more helpful than the route itself.
What Kind of Models Power Predictive Freight ETA Systems?
Different shops take different approaches. Here’s the general breakdown:
Traditional Models
- Gradient Boosted Trees (like XGBoost or LightGBM): Great for structured data. Fast to train. Easy to tune.
- Rule-based layers: Some things are still easier to hard-code—like “add 45 minutes if it’s a Friday before a holiday.”
More Advanced Setups
- RNNs or LSTMs: Good when you need to consider sequences, like stops along a route.
- Temporal models: Newer techniques that juggle time-series inputs better.
- Geo-embedding tricks: These help the model understand locations in a smart way—not just lat/long points.
Do you need all of this? Depends. Some carriers get great results with simple stuff and clean, real-time freight data.
The Data and Infra Behind the Scenes
Now we’re getting into the messy middle—the plumbing that makes the whole predictive ETA engine tick.
Real-Time Data Matters
For something like this to work, you need fast data. A system like Estuary (a platform that handles real-time pipelines) is a lifesaver here. It helps you:
- Capture changes from databases (CDC-style)
- Pull GPS and API data in real time
- Clean and re-shape it into model-ready features
- Route it to wherever it’s needed—model inputs, dashboards, or both
And bonus: it handles schema changes automatically, which, if you’ve been burned by that before, you know it’s a big deal.
Feature Building & Training in Freight Logistics
- Stream processing tools like Beam or Spark are handy for real-time joins or rolling averages.
- Schedulers (think Airflow) help you prep features on a cadence.
- Feature stores? Some teams use Feast, others just build their own on something like Iceberg.
- When it’s time to train, you’ve got options: scikit-learn for quick iterations, TensorFlow or PyTorch for deeper work, and cloud platforms like Vertex AI if you want managed compute.
- For serving predictions, FastAPI + Redis does the trick.
Where Predictive ETAs Get Tricky
Everything sounds great until… it isn’t. Here’s what trips people up:
- GPS dropouts – You’d be shocked how often a truck goes dark.
- Cold starts – New routes, new drivers… the model hasn’t seen it yet.
- Time zones – Still a pain. Always double-check your offsets.
- Gaps in training data – Even small holes can mess up long-haul predictions.
- SLAs vs. models – Business expectations don’t always match what the model can realistically predict.
These aren’t blockers, just reminders that freight engineering is always messier than the diagram.
The Results of Getting Predictive ETAs Right
When teams get this right, the payoff shows up fast.
- Yard operations get smoother.
- Fewer angry “where’s my truck?” calls.
- Load planners make better bids with more confidence.
- Carrier performance gets easier to track and compare.
It’s not just tech for tech’s sake. It’s the kind of change that hits both the top line and bottom line.
What’s Coming Next in Freight ETA Technology?
The freight world isn’t standing still. Here’s what’s coming up:
- Federated learning – So models can learn from multiple carriers without sharing raw data.
- Edge predictions – Think: models running on telematics devices themselves.
- Self-updating ETAs – Systems that learn from their own misses and adjust on the fly.
- TMS integration – Predictions that automatically adjust scheduling and planning tools.
We’re inching closer to a world where Freight ETAs aren’t just passive guesses—they’re active parts of decision-making.
Wrapping It Up
Predictive ETAs aren’t a luxury anymore—they’re a basic need in modern freight logistics. The good news is that the tools are ready, the data’s already flowing, and the models are more brilliant than ever.
But the way you build it still matters. It all adds up from data ingestion to model serving, from edge cases to fallback logic. Carriers and logistics teams that invest in this today won’t just improve delivery accuracy. They’ll build systems that learn, adapt, and get sharper with every load.
And maybe, just maybe, we’ll all stop wondering when that shipment will get here.
Ready to Build Predictive Freight ETAs That Actually Work?
Want to explore how to go from static ETAs to intelligent, real-time predictions? Connect to a Real-Time Data Expert
Want to see how real-time freight tracking works in action? Check out our deep dive on Estuary + Tinybird for logistics dashboards.
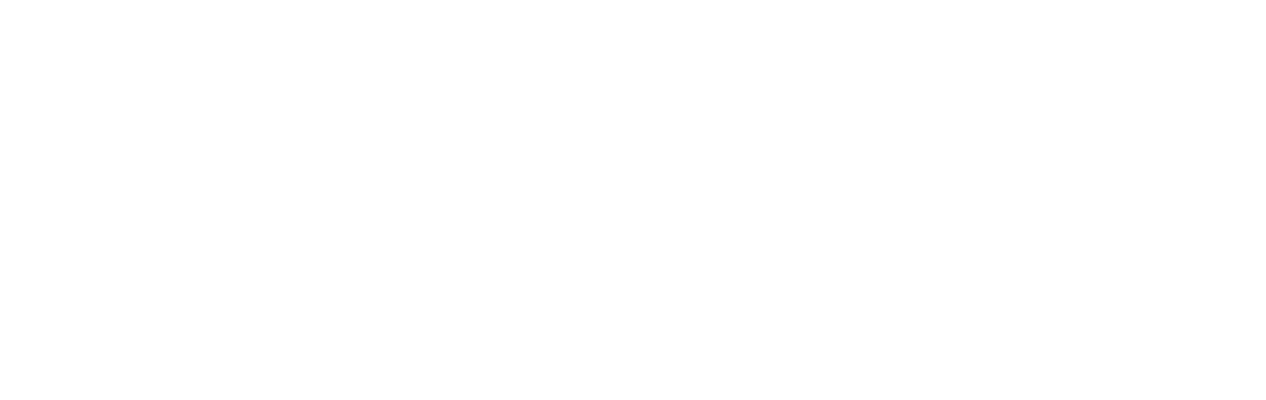
About the author
Dani is a data professional with a rich background in data engineering and real-time data platforms. At Estuary, Daniel focuses on promoting cutting-edge streaming solutions, helping to bridge the gap between technical innovation and developer adoption. With deep expertise in cloud-native and streaming technologies, Dani has successfully supported startups and enterprises in building robust data solutions.
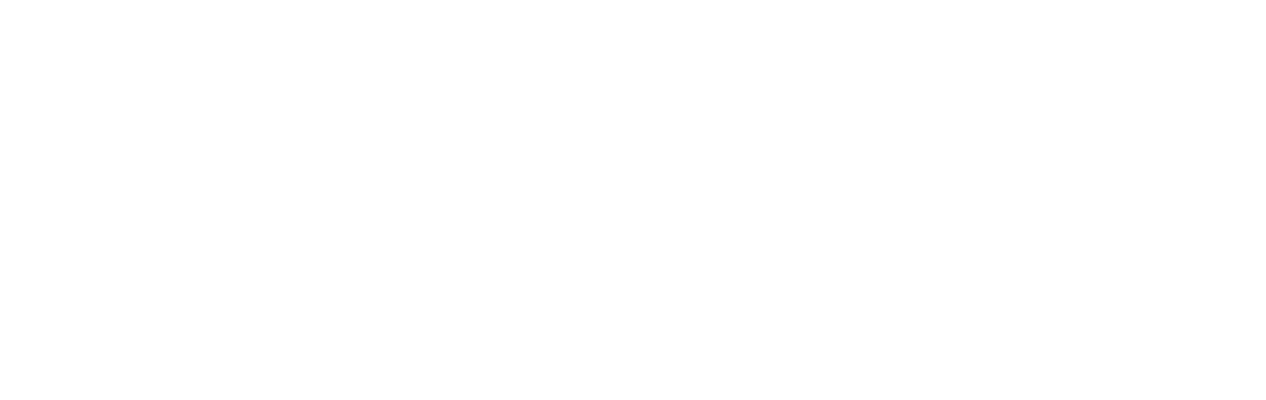